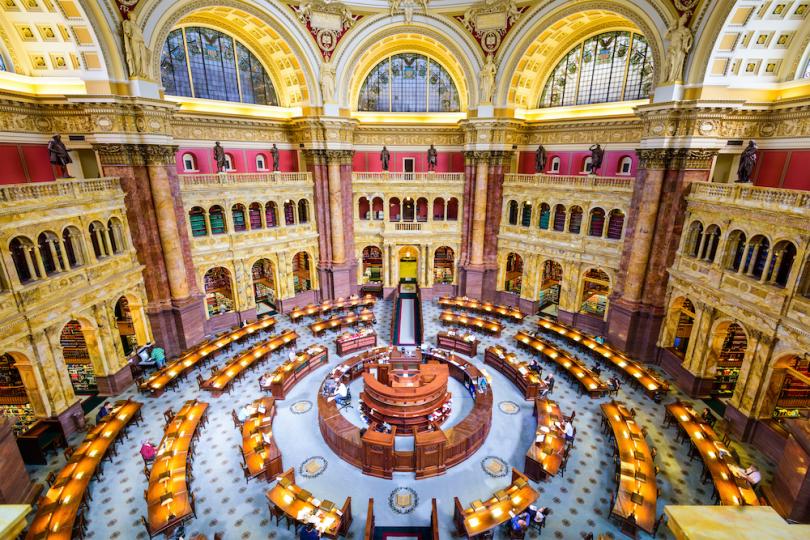
The Library of Congress captures the essence of big data. Each collection within the world’s largest library — which contains some 170 million items and growing — is a dataset waiting for analysis. But just like with other big data undertakings, siloing gets in the way.
Consider the library’s massive collection of newspapers. One research fellow hoped to use the Chronicling America collection — millions of digitized newspaper pages, dating from 1789 to 1963 — to compare historical classified ads against contemporary Craigslist posts, to see how patterns of fake ads have taken shape over time. She even signed up for a class to learn Python to pull it off. But it turned out there was no effective way to drill down by category and subset the ads from the bulk of the newspaper text.
A similar problem played out for a graduate student who hoped to use the library’s Wayback Machine-esque web archive of election campaign sites, in order to see whether candidates changed positions after an event. But since crawlers build Web ARChive files by time of site capture, without thematic consistency, queries returned prohibitively large amounts of unrelated data. Plus, files just get massive overall.
“Before a researcher works with web archives, they need to do some significant data reduction,” said Kate Zwaard, director of digital strategy at the Library of Congress “And it’s not easy for us to transfer these many-terabytes-large collections to researchers. It costs money.”
Collection interfaces are often built item-level, without a window on the whole.
“But if we’re thinking about presenting collections and data at scale, we need to be completely reconsidering a different model,” said Meghan Ferriter, senior innovation specialist at the Library of Congress.
While taking stock of such access obstacles, the library’s digital strategy wing is also asking, what exactly are the kinds of topic focus areas and technical approaches that best dovetail with research that can only be done by analyzing large-scale data sets, via machine learning.
To that end, the Library of Congress put out a call for proposal late September — an agency announcement looking for up to four researchers “to experiment with solutions to problems that can only be explored at scale.” Another call will go out later this winter for a technical contractor who will focus on the kinds of data transformation challenges described above.
Researchers, who will be in residence from May 2021 through January 2022, will document their individual work and share regular updates. Data artist Jer Thorp will be documenting the project as a whole.
The project, called Computing Cultural Heritage in the Cloud, aims to create a virtuous cycle of modeling approaches and complementary data wrangling to advance machine learning research techniques within the library, and for the so-called GLAM (galleries, libraries, archives, museums) sector more broadly.
“We’re trying to think about, ‘How do we create a comparable model for those who want to research with digital collections at a broader scale or look across our research collections?’” said Ferriter.
Another target of the project? Reusability. Even when researchers do the hard-slog work of cleaning data for a specific use case, the library currently doesn’t have a great way to reinstitute that cleaned data set back into its collection for future use. That will hopefully change.
“If we have a preliminary set of data transformation that’s a stepping off point for more specific questions, we could then try to host that, and we plan to do that as part of this project,” Fettiter said.
RelatedBuilding a Machine Learning Pipeline? Here’s What You Need to Know
Photo: ShutterstockCollections As Data
Digitizing library collections is nothing new. The Library of Congress has been doing so since 1993. It also has a few APIs that allow for subsetting of some smaller data sets. The concept of collections as data, however, is more recent.
To a growing extent, institutions like the Library of Congress have made digitized or natively digital collections, and their attendant metadata, available explicitly for computational analysis. But many collections weren’t digitized in a way that centered a big-data analytics lens. So museum and library archivists have had to come together in recent years in order to hash out the best framework.
Last year’s “Always Already Computational: Collections as Data” report, co-authored by Laurie Allen, a program analyst at the Library of Congress’ Office of Digital Strategy, covers topics ranging from advice on full-text collection inventories — “document rights status, license status, discoverability and downloadability” — to noting characteristics that make a digital collection a good collections-as-data candidate — thorough metadata, strong optical character recognition results, popularity and relevance to other projects.
At the same time, the report also underscored that the concept remains a work in progress. “There’s no clean, shared state of play across collections,” Ferriter said. “Has that changed? I think the answer is no. But our awareness about it has changed. And people are thinking and speaking through the lens of collections as data a lot more.”
That “foundational work” nonetheless helped guide the Library of Congress’ project, most notably with collection cover sheets, Ferriter said. It’s basically the prep before the prep — documenting what collections contain as extensively as possible to make future data transformation easier.
Staff has identified some collections that have strong data-analysis potential and begun detailing cover sheets for each, outlining information about format, size, rights issues — anything that might aid future research on that collection.
“For computational uses especially, researchers want to understand the structure of the collection at multiple levels, as well as its provenance — where it came from and how it has been transformed, as well as what is missing from the data, and what kinds of uses are allowed with it,” Allen wrote in January in a blog post that included an early-stage sheet template.
Collections with prioritization potential include those that aren’t rights-restricted and those that have historically proven fruitful for researchers. Examples include digitized versions of stereograph cards and the Sanborn maps, which draw a picture of 19th and 20th century architecture and city planning and have been used to help understand changes within given settings. But the full range will be influenced by the research topics mapped out by the four researchers to be hired.
RelatedImproving Racial Equity in Data Integration
Photo: ShutterstockSlow and Steady… and Ethical
The Library of Congress is far removed from Silicon Valley geographically, and also temperamentally. A report on the state of machine learning in libraries, commissioned by the Library of Congress and published in July, draws a contrast between the “move fast, break things” ethos that defined Big Tech and the methodical approach favored by librarians. That has positive implications for things like setting standards before adopting new technologies, but it’s valuable for larger ethical issues, too.
That paper, and other research conducted or supported by the Library of Congress, foregrounds the dangers of algorithmic bias far more directly than is often the case in the commercial sector. As the report notes, even though the capacity for harm within research institutions is far lower than in use cases like credit lending and gauging recidivism likelihood, narrow or biased data sets could produce flawed research or “inadvertently echo and amplify outdated or even offensive subject terms.”
Clear value statements and attention to organizational transparency and staff data fluency are key, but so are more granular details, like establishing — and making readily available — ground truth data sets and benchmarking data, researchers noted. That means, for image data, having metadata that doesn’t just explain what is pictured, but considers a host of possible features, like “digitization source, contrast, skew, noise, range effect, complexity (or a difficulty measure of some sort).” As the report underscores, the technical concerns are inextricably linked with social ones.
Such level of concern tracks with libraries’ larger track record of prudence, said Zwaard.
“There’s a long, well-developed history of professional ethics,” she said. “Libraries have been serving sensitive materials for centuries and have a really good understanding of what’s appropriate.”
The project, whose advisory board includes equity advocate and Algorithms of Oppression author and Safiya Noble, advises applicants to propose projects “that are diverse in terms of their topics, their approaches, and the required collections.”
Of course, machine learning’s great promise in cultural heritage research — both broadly and within the Library of Congress project — is that it might reveal connections and insights that are inadvertently hidden within the stacks.
“One of the most exciting things about looking across collections at scale is that we can see stories and surface patterns that were not as available, or not as prominently presented to the public,” said Ferriter.
RelatedSynthetic Data: How AI Uses Fake Data for Genuine Gains
Source: https://builtin.com/machine-learning/library-congress