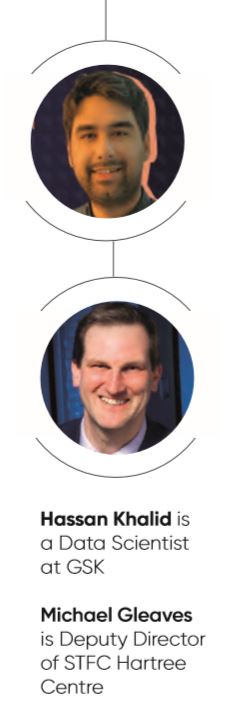
During an interactive discussion session, Michael Gleaves and Hassan Khalid revealed the most suitable industrial applications for artificial intelligence and ways to achieve a true return on investment.
Where to start?
Michael Gleaves: Attaching simple and affordable sensors to machines is a good first step towards engaging with machine learning (ML) techniques. Once you have those streams of data, then you can begin identifying patterns and trends and start to optimise your asset usage.
Ultimately, sourcing quality, unbiased data is paramount; without that, you can’t begin to think about how to extract value from those data streams.
What skills do you need?
Hassan Khalid: Technical knowledge is important, but don’t underestimate domain knowledge. Somebody who has spent years on the manufacturing line might be able to tell you a lot that you’re missing from your data.
Similarly, don’t underestimate software engineering. A lot of things go unnecessarily wrong, because of software engineering lapses. People who come from scientific backgrounds, like me, have to train in software engineering skills to make sure that we’re implementing what we think we’re implementing.
Most companies adopt a ‘develop, test, explore’ cycle, so having somebody internally to run that cycle is important. Having AI and ML experts feeding hypotheses for your domain experts to test in a virtuous loop is equally important.
MG: If you’re going to disrupt parts of your business with these technologies then it’s beholden on you to have a small team of specialist skills that can adapt those technologies and use them to drive your business forward. Also, it’s better to have some level of internal capability within your organisation so you can make intelligence purchasing decisions.
Manufacturers should also consider attracting and retraining physicists and astronomers to use these tools. They have the right mindset and they’ve generally coded as part of their PhDs, so they should be able to be converted into these roles relatively easily.
How do you attain executive buy-in?
HK: This is often the most difficult part. It helps to align your ideas and projects with your organisation’s broader data or digital strategy. If a particular experiment isn’t aligned with that strategy, then it’s probably not the best idea to spend too much time or resource on it.
MG: We’ve started to apply a design thinking approach to the user requirements gathering. So, taking out the intended technology or technique and focusing specifically on the business problem, the use case, and exploring what happened before, what happened afterwards and how that would be used within the business.
It’s really critical that you pick good projects to start with because that creates momentum and continued investment. But at some level, there has to be a leap of faith that you think a particular technology has value and you’re willing to invest in it.
How can AI and ML be used for predictive maintenance issues?
HK: From a practitioners perspective, predictive maintenance is challenging because most of the time your data doesn’t contain examples of what failure looks like. When you don’t have negative examples, it’s very difficult to train your system.
We’ve conducted video and live-image analyses, as well as noise-based analysis where we’re looking for changes in vibrations. The opportunities are essentially endless, the key question is whether the data is giving you a real picture of failure to learn from.
MG: Techniques such as using pattern recognition to identify types of failure that happen within a period of time and then working to schedule that to routine maintenance have been used significantly.
An area of increasing interest is the using the data processing and logs sitting in SCADA systems to identify patterns of failure as well. Those efforts can be aided by digitising human logs and add natural language processing to provide additional context.
Are AI and ML realistic propositions for SMEs?
HK: These things can become expensive and there’s a lot of hidden tries so expect failure. I think you can pull it
off on a tight budget, but you’ve got to compensate that with time. It’s unrealistic to think you can spend the minimum amount of time with the minimum amount of investment.
There’s a lot of open source technology, code, publications and explanations available, meaning most of the work has been done. For organisations starting on this journey, they need to think very hard about their data strategy. Adding a machine learning strategy to that data foundation becomes slightly simpler, in relative terms.
The most expensive thing for an SME would be the talent itself, and that’s where SMEs are competing with large organisations. Then again, given ownership and the flexibility to work, SMEs shouldn’t have a problem getting top talent away from the giants.
MG: We have done a lot of work with SMEs, particularly in the Liverpool City Region through the LCR 4.0 programme (lcr4.uk) where we’ve been able to demonstrate use cases that extract data from a particular piece of equipment or business process and then process that data to generate a benefit.
What’s the best coding language to learn for AI and ML projects?
HK: For SMEs, Python has taken the leap in terms of open source development so provides access to good tutorials and a lot of prepared solutions. If you deal with an Amazon level of data, then you may need to consider the added capabilities offered by Scala, SPARK and Julia.
More information www.hartree.stfc.ac.uk
Header image courtesy Deposit Photos