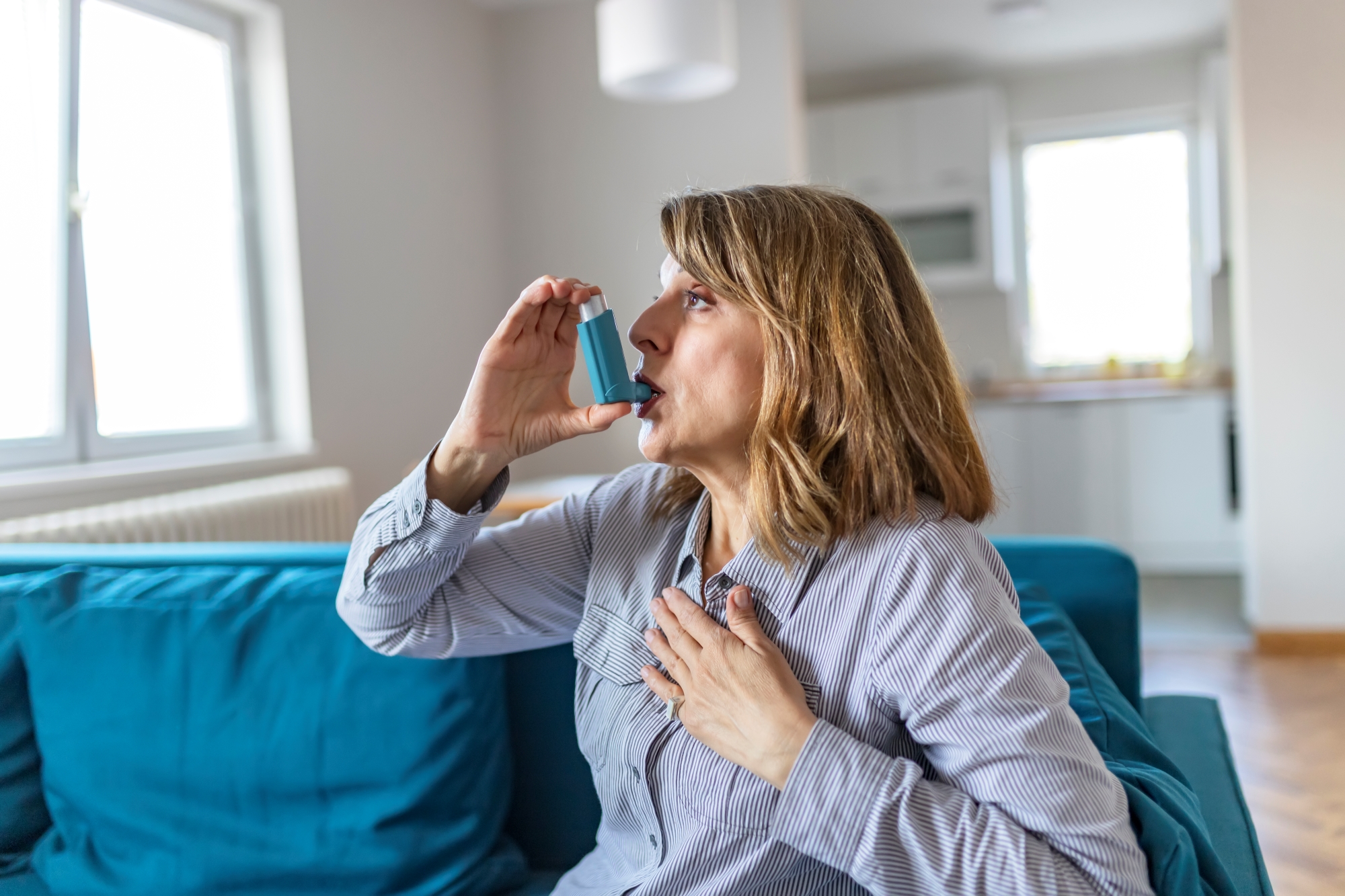
A novel machine learning algorithm using real-world outpatient data from electronic health records (EHRs) was capable of predicting asthma exacerbation risk, according to study results published in CHEST.
Researchers from the Cleveland Clinic analyzed deidentified EHR data of 60,302 patients with asthma (median age, 47.5 years) who were followed at the institution from 2010 to 2018 (median follow-up, 3 years). Input variables for the machine learning models included demographic information, comorbidities, laboratory values, and use of asthma medications.
The investigators built 3 different models with logistic regression, random forest, and the Light Gradient Boosting Machine (LightGBM) decision tree algorithm. These models were built to predict nonsevere asthma exacerbations that required oral glucocorticoid burst, emergency department (ED) visits, and hospitalizations among patients with asthma.
A total of 19,772 (32.8%) patients from the EHR cohort had at least 1 nonsevere exacerbation that required an oral glucocorticoid burst. Furthermore, 1748 (2.9%) patients required an ED visit and 902 (1.5%) patients required hospitalization.
Continue Reading
The algorithm that best predicted nonsevere asthma exacerbations, ED visits, and hospitalizations was the LightGBM, which featured an area under the receiver operator curve of 0.71 (95% CI, 0.70-0.72) for nonsevere exacerbations, 0.88 (95% CI, 0.86-0.89) for ED visits, and 0.85 (95% CI, 0.82-0.88) for hospitalizations.
Risk factors for nonsevere exacerbation, ED visits, and hospitalization included age, long-acting beta agonist use, and high-dose inhaled glucocorticoid or chronic oral glucocorticoid therapy use. Meanwhile in 9448 patients with spirometry data, the top risk factors for asthma exacerbations, ED visits, and hospitalizations included low forced expiratory volume in 1 second (FEV1) and FEV1/FVC (forced vital capacity) ratio. The addition of pulmonary function tests to this model, however, did not lead to an improvement in the models’ prediction power.
A limitation of this study was the reliance on EHR data from a single center, which may not be generalizable across all centers and may have missing clinical information.
The researchers suggested that models built with machine learning algorithms that incorporate real-world EHR data can also “be incorporated into clinical decision tools to enhance outpatient care and prevent adverse outcomes.”
Reference
Zein JG, Wu C-P, Attaway AH, Zhang P, Nazha A. Novel machine learning can predict acute asthma exacerbation. Published online January 10, 2021. CHEST. doi:10.1016/j.chest.2020.12.051
Topics:
Asthma
Source: https://www.pulmonologyadvisor.com/home/topics/asthma/machine-learning-models-using-ehr-data-may-predict-asthma-exacerbation-risk/