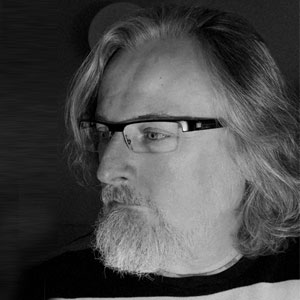
Stuart Curley, CTO, NGA Human Resources
Everyone seems to want artificial intelligence (AI) and machine learning (ML), but few can define exactly what it is they want from it! While business leaders have high expectations of AI and ML, often, after months of optimistic experiments, proof of concepts, and pilots, they are left disappointed by the results.
Why are Business Leaders Disappointed?
Why? Because despite media hype, AI and ML are not about to sweep in and immediately solve all our business challenges. They’re not silver bullets. Artificial intelligence and machine learning present evolutions in technology processes. Success comes from quality data—lots of it, managed by highly qualified data scientists.
We need to set expectations. Match what organizations hope to achieve by applying artificial intelligence and machine learning, against what is currently realistic. Against this, we must then line up the hurdles that must be leapt over to get to the best results.
Experience Tells Us…
Having spent several years working on different proof of concepts, with different artificial intelligence and machine learning technologies, we have learnt important fundamental lessons. There are three key areas of capability needed to be successful with artificial intelligence and machine learning. These
1) People Skills and Experience
Machine learning is dependent on complex mathematical calculations. This means we need data scientists who understand statistics and the maths that underpin both artificial intelligence and machine learning.


The areas of maths include linear and logistic regression. Both statistical models are used to describe data and to identify the relationships between variables. To apply these statistical models to supervised learning, support vector machines are used. A human will find this extremely difficult to do manually. There are other methods too, such as linear discriminant analysis.
While this may seem obvious, many organisations start by experimenting with artificial intelligence and machine learning tools. Most often, these fail to deliver the expected results and there is huge disappointment.
2) Artificial Intelligence and Machine Learning Technology Experts
AI and ML technology can seem like a bewildering Swiss Army Knife. Lots of different tools, each with very specific purposes. Each tool requires an expert who can use it for its purpose and in the right sequence.
There are many who claim to have artificial intelligence and machine learning technology. Mostly the DIY experts. To complete the analogy, they are more likely to use a hammer to drive a screw in, with equal success.
On top of this, the major vendors are in a neck-and-neck race to constantly improve their toolset. The rate with which artificial intelligence and machine learning technology is developing is extraordinary. This makes it almost too easy for DIYers to start a project using these tools. And, as new versions come out, for costs to spiral as they become more and more dependent on the tools. Few will achieve great results.
I strongly recommend that you consult real experts on the toolsets you need for before investing in a potentially bottomless pit.
3) Domain Knowledge Experts
Again essential, these people can really explain the meaning of the data in the domain. Where the technology experts help us with the tools and the data scientists with getting the best results from our data using machine learning techniques, domain knowledge understand the specific data fields. It is therefore critical that someone is freed up from business as usual to work with the other experts.
AI and ML Need Good Data, and Lots of it
Many AI and ML initiatives are working on gigabytes of data. To get good results, terabytes or even petabytes are needed. Huge volumes of data are essential for the statistical models to produce good results.
This data must also be of good quality and as complete as possible. It is then trained. A well-known machine learning experiment tries to recognise individual cats from millions of photographs. While the cats in the training machine are in all kinds of positions, they are complete, that is, they do not have chunks of the cat missing.
To the contrary, enterprise data often has empty fields (chunks missing) or fields with incorrect data (heads where legs should be). This makes for a poor set of training data. If you cannot train your machine well, that is, by using high volumes of complete data, you can never get great results.
Experience shows that the preparation of a good set of training data is key to good outcomes from machine learning experiments. This means often the need to invest in improving your dataset. It is resource intensive to clean and improve the data. Without this investment, you are very unlikely to reap the full potential of machine learning.
Best Results Come From Hard Work and a Scientific Approach
While everyone wants a piece of the artificial intelligence and machine learning pie, as I said from the start, it is not a silver bullet. It requires hard work and a highly sophisticated scientific approach. There are plenty of snake oil sales people ready to promise you the earth, but there really are no shortcuts.
To avoid wasting your time, money, and internal reputation, start by bringing together people who really know what they are talking about. And treat them right—they are scientists. Experts in their very niche field. While it is difficult to wait this longer, considered approach to the integration of AI and machine learning into your organization will eventually be the fastest route to success.
Check Out: Top Artificial Intelligence Companies