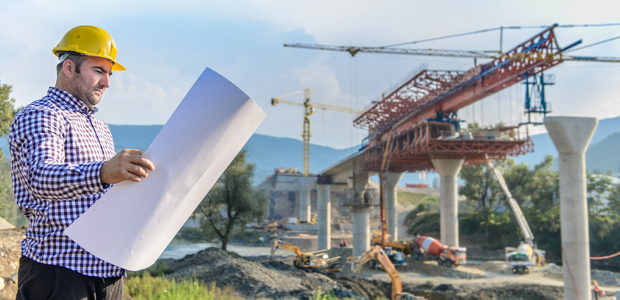
Machine learning helps Indiana DOT bundle projects
By Stephanie Kanowitz
Apr 22, 2021
The Indiana Transportation Department (INDOT) is applying machine learning (ML) to project bundling to maximize cost savings and reduce the time it takes to create bundles from a week to minutes.
Transportation engineers typically group infrastructure construction projects into bundles to gain efficiencies in contracting and project management, minimize infrastructure disruption and achieve economies of scale. Bundles are created from similar projects – bridge projects, freeway lighting installations or safety improvements – or from a variety of types of work in a specific location. Bundling is complex, manual, subjective process, with engineers usually working from maps and spreadsheets.
INDOT has done manual project bundling for about five years and used that data to test benefits of ML-enabled bundling, in which an algorithm that had been trained on historic data makes bundling suggestions. Using the technology has increased savings from bundling by 40% and could save INDOT $108 million in the next four years, according to the Federal Highway Administration.
But the real advantage is speed, said Louis Feagans, managing director of asset management at INDOT.
“Instead of having to sit down and create maps and drawing everything by hand,” Feagans said, ML speeds the process. “What used to take us over a week to develop … now it’s a day at the most.”
After determining what projects are candidates for bundling, INDOT – which evaluates more than 1,000 projects for bundling each year – uploads the data from its project maintenance database, the Deighton Total Infrastructure Management System (dTIMS), into the ML platform, built by Foro, a Group Solutions company. INDOT runs the algorithm once with the initial criteria, such as the number of miles the project can span or the location, and reviews the suggested bundles.
“We go through all the results and can accept,” Feagans said. “‘Yes, we like these bundles; these make sense to us,’ [or] ‘Hey, this one, due to contractor availability or due to other issues, we don’t like this,’ and so we won’t accept that one.” After whittling down the possibilities, they retest the bundles against additional parameters, such as adding five miles to the distance to be included.
Foro trained the platform on INDOT’s manual bundling data from projects scheduled for fiscal years 2021-24 and a data dictionary of project data fields, business rules and decision criteria, said Brett Boston, the company’s managing partner. Data elements from dTIMS included estimated project cost, midpoint of each project’s location, the corridor number and whether there was a county union affiliation.
“That gave them the ability to look at what [ML] would have done for those previous four years vs. what they did, and our bundle found $120 million in additional savings on top of what they had already saved,” Boston said. Plus, it reduced the amount of staff time required to produce contract bundles by 1,400 hours.
Foro also built a data translation tool so that whatever dataset the department provides, the platform can import and standardize it.
“It’s almost an [application programming interface] that’s between their planning tool, that’s an in-house tool, and our software,” Boston said. “They can give [data] to us in whatever format, we automatically change it to what we need and then we give it back to them in the exact format that they need to load their internal systems,” he said.
INDOT engineers manipulate the bundling options through a dashboard, where they can make changes to, say, the radius or road in need of work and automatically see how those adjustments affect the overall project. What’s more, they can view all the projects on a map.
“This can take the hard work out of the process, and the hard work is having a bunch of people sit down and try to figure out all these bundles — let the machine do that,” Boston said. With that preliminary work done, state engineers can get creative running what-if scenarios using the software.
Previously, INDOT used maps to try to look for bundles. Besides being time-consuming, “we had missed opportunities” because projects typically didn’t cross the boundaries of the state’s six districts, Feagans said. With ML, “we’ve eliminated the Great Wall of China,” because projects easily cross district lines.
On April 16, INDOT started using the platform to gain more efficiencies by jointly bundling with local metropolitan bundling authorities, planning organizations and cities, Boston said.
This summer, Feagans plans to tweak the platform again to search for bundles based on multiple years.
“I want to look at a three- or four-year window and give them some new business rules and say, ‘OK, you can move this project plus or minus four years, road jobs one or two years,’ and then give them that four- or five-year window for that project,” he said.
This will help with maintenance planning, Boston added. For instance, if in fiscal years 2026-28, several projects will come due for the same corridor, the platform can help the department bundle those efforts so that traffic doesn’t have to close to a given area two years in a row.
INDOT’s use of ML for transportation project bundling is the first of its kind, according to a report from Foro, but it’s highly replicable.
“The challenge will be cultural,” Boston said. “Adopting machine learning really has an impact on your actual contracting process. Every piece of software you add to a business changes the way the business is run.”
About the Author
Stephanie Kanowitz is a freelance writer based in northern Virginia.
Source: https://gcn.com/articles/2021/04/22/indiana-dot-ml-bundling.aspx