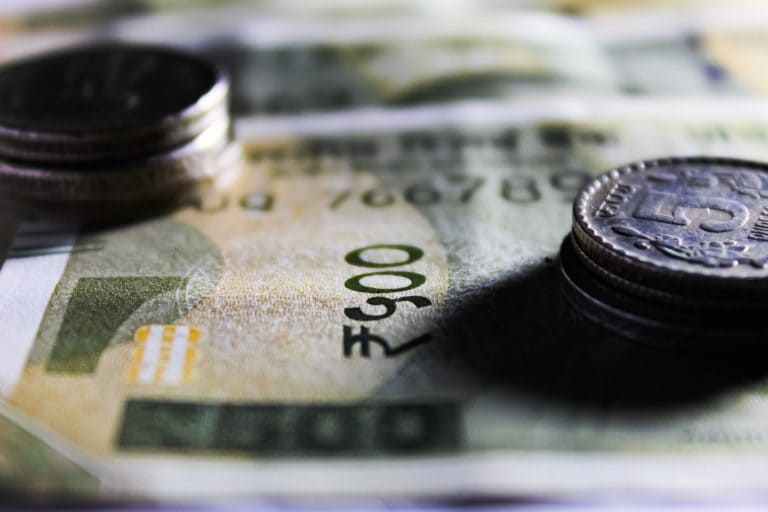
Technologies such as artificial intelligence and machine learning models that were once considered nascent and on the horizon are now at the forefront of financial services, especially lending. Hesitation to adopt these technologies could prove costly for lending institutions because of the game-changing advantages they bring to the business model.
Here’s a quick dive into the lending business to understand more:
36 percent of large financial institutions are already investing in these technologies, and almost 70 percent report that they are planning to in the near future. – Emerj
Compared to the global landscape, India’s financial services sector has thrived in the past decade. Much of this growth is owed to the rapid digitization of data and data-related products and services. India’s digital thrust was made possible by various public and private collaborative initiatives, many of which were led by India’s Union Government and industry bodies.
The resultant outcome has shifted India many places forward in the global digital competitiveness index and according to a McKinsey report. Over 80 percent of individuals now own a bank account, in contrast to just about 20 percent a decade ago. Financial inclusion of unbanked businesses and people has more than doubled over the period 2010-2017, according to a World Bank research report.
While there has been plenty of headway in terms of financial inclusion, this progress was made on a small base. There remains a much greater set of businesses outside the formal credit ecosystem, for whom access to capital remains the biggest hurdle to economic progress.
Economic growth slowed down in recent years and it received a massive jolt owing to the ongoing COVID-19 pandemic. As production sputters along, banks and financial institutions that have fed liquidity into the system up to this point need to find new avenues to sustain healthy growth. The modernization of business technologies and the adoption of artificial intelligence algorithms and machine learning models may be the formula lenders are missing. Let’s consider a typical lending cycle to see how automation using AI and ML predictive data models can alleviate challenges currently plaguing the system.
Lending as we knew it
In any loan disbursement program, there are several processes that need to be carried out by stakeholders at various stages and in different capacities. Loan lifecycle management is resource-intensive and can take long periods of time.
It includes:
1. Aggregating loan applications from affiliated or externals parties
2. Onboarding and verification which includes processes like KYC (Know your customer), AML (Anti-Money Laundering), CDD (Customer Due Diligence), NL (Negative List), BL (Black List), Pending litigations checks
3. The underwriting process
4. Approval and disbursal
5. Monitoring borrowers to pre-empt delinquencies or defaults
These processes incur significant time and manpower costs because of the many steps involved – from the initial phase of screening prospects to making the final lending decision, managing the loan disbursals and finally, monitoring the portfolio.
New-age technologies coupled with alternative data sources make it possible to automate a wide variety of tasks and processes which require high operational bandwidth. For every step of the way, there is a more efficient and reliable alternative using digital automation aided by AI/ML tools. The advantage is not just in terms of cost reduction but also in terms of process efficiency and customer experience. Here’s a look at how automation and explainable AI can change the face of lending in the 21st Century.
Seamless Onboarding and Digital Trust
Today sophisticated tools allow lenders to digitally onboard customers by aggregating applications and prepopulating authenticated data from multiple channels. These customer profiles are put through an initial decision-making model that has the requisite checks and balances in place to provide sound credit decisions. This digitized process can help streamline the loan application screening process and can also help identify the probable bad apples without bias in the first step of the journey. Identifying suitable customers is possible by deploying a recommendation engine that filters and matches prospects with different financial products.
Agile software platforms equip business experts with the ability to standardise these decision models with clear business logic in the form of rules. These models are augmented with machine learning capabilities to enhance decision-making by continually learning through sustained data being fed to the system. Hence, they reduce the possibility of human error and speed up the process.
And finally, the task of collecting a customer’s residual documents is done by getting them to directly upload these to the platform. Overall, customer onboarding can now be done safely and remotely in a matter of minutes (sometimes as low as 90 seconds) as opposed to weeks of running around and via bureaucratic paperwork.
Risk Assessment and Underwriting via Automation
Based on the data acquired from borrowers, credit scoring algorithms can be tailored specifically for financial institutions based on their domain expertise, size of loan book and risk appetite. This algorithm can also be enriched by integrating alternative data points about borrowers, such as employee data (from EPFO) and news sentiment analysis. The scoring models are also used to evaluate the risk of associating with groups of connected promoters or directors as well as broader industry parameters such as regulation or consolidation.
The workflows of underwriting the loans and analyzing collaterals and assets can also be automated. The dynamic nature of the value of these collaterals can be encompassed by feeding the data of the market pool into the system that continually analyses, learns and provides risk-adjusted insights. Alternatively, lenders can switch to cash flow-based lending, which is based on recent business performance. This has proven to be better for some lenders when it comes to reducing their NPAs and loan defaults.
Monitoring and Collections with Explainable AI/ML models
One of the most overlooked aspects of the credit management lifecycle is monitoring. With the advent of AI/ML technology, data gathering and analysis can be performed at a much greater pace compared to the conventional process. AI algorithms can be configured to monitor the financial and non-financial parameters of all borrowers in the portfolio. These systems raise early warnings when the possibility of risk has aggravated due to tangible inputs. This gives financial institutions better opportunities to course correct. Risk officers or analysts can trigger a request to their collections department to resolve a problematic situation with a borrower before it’s too late.
The latest AI/ML tools also give lenders the power to automate a host of tools in their collections modules. This saves time, resources and enables lenders to focus more on the accounts that need emphasis with foreclosure, debt restructuring or an early write-off if required.
Conclusion
Automating the credit management lifecycle with explainable AI and ML tools inevitable for financial institutions. It improves the process efficiency and customer experience, reduces turn around time, provides better compliance, reduces operating costs, and enables financial institutions to be proactive in their approach. The long chain of cumbersome paperwork and individual-dependent decisions can be avoided by adopting automation. These tools also make it easier for lenders to move to flow-based lending rather than persisting with asset-based lending models.
The writer, Meghna Suryakumar, is the Founder and CEO of Crediwatch. The views expressed are personal
Source: https://www.cnbctv18.com/finance/lending-enabling-effective-credit-lifecycle-management-using-ai-and-machine-learning-9259431.htm