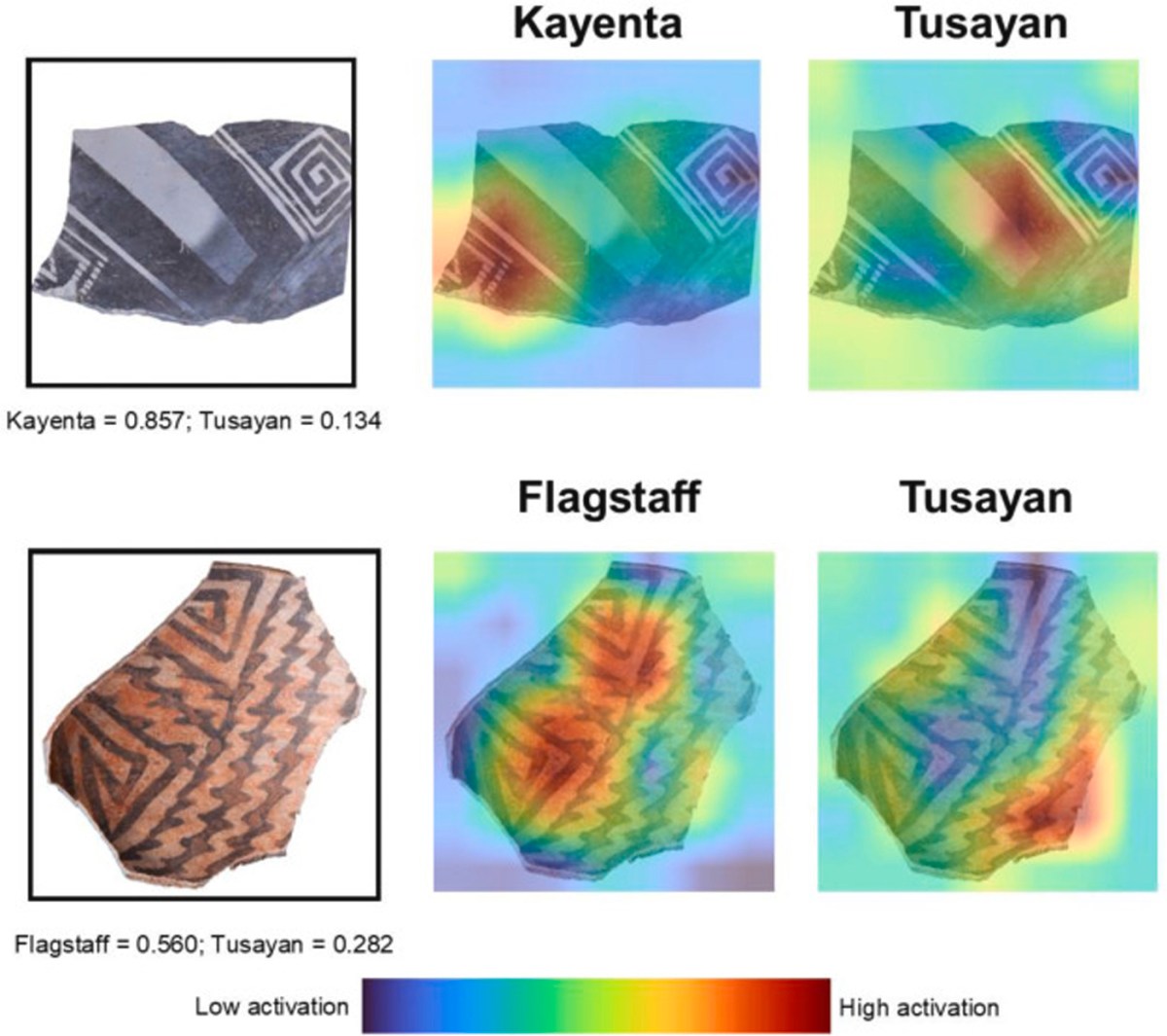
Support Hyperallergic’s independent arts journalism.
Grad-CAM heat maps for TWW sherds, showing areas of high (red) and low (blue) model activation for a Kayenta sherd (top) and Flagstaff sherd (bottom). CNN-model-calculated type confidences shown below each sherd. (all images courtesy the University of Arizona Department of Anthropology)
In our age of innovation, it seems that every week brings new machine technology able to engage in practices formerly relegated to human evaluation. A recent publication in the Journal of Archaeological Science (Vol. 130) presents research from Northern Arizona University’s Department of Anthropology, where scientists have succeeded in teaching computers to perform the complex task of rapidly sorting thousands of fragmented pottery designs — called sherds — into differing stylistic categories.
When properly trained, the technology “can assign types to digital images of decorated sherds with an accuracy comparable to, and sometimes higher than, four expert-level contemporary archaeologists,” write researchers Leszek M. Pawlowicz and Christian E. Downum, in the abstract for Applications of deep learning to decorated ceramic typology and classification: A case study using Tusayan White Ware from Northeast Arizona. The researchers continue to say that the deep learning model can match an “unclassified sherd image” to its counterparts by sorting through thousands of digital images.
Sherd images with diagnostic design elements of Tusayan White Ware types identified.
As the title suggests, the study focused on sherds from fragments of Tusayan White Ware, a type of hand-formed painted pottery often found in Northeastern Arizona, used mostly for serving food and transporting and storing water during the Pueblo I through Pueblo III time periods (approximately 825–1300 CE). Vessels typically have geometric designs rendered in dark brown and black organic paint on white backgrounds. Researchers assigned the task of using digital photos of thousands of sherds to sort styles to human experts, and compared the results to those of Convolutional Neural Networks (CNNs) given the same assignment. Motifs include the checkerboard-style “Black Mesa”; the “Dogoshzi” wings and curves filled with diagonal lines; and at least a half-dozen other known typologies that feature discernible differences in brushstroke, line width, and decorative fill variations.
Obviously, the ability to accurately and rapidly classify the thousands of small artifacts offers huge potential benefits to archaeologists and researchers who excavate numerous such sherds in pursuit of bigger artifacts. Each of the four participants in this study (including Downum, one of the authors) has “30 or more years of field and laboratory analysis of ceramics, and each during their careers has personally classified tens of thousands of TWW potsherds,” according to the study methodology. These experts classified an initial set of 3,064 sherd photographs, and the CNN findings were extremely encouraging by comparison.
“The CNN model achieved an accuracy on the consensus dataset comparable to that of experienced human classifiers, comparing favorably to previous studies,” said the study’s conclusion. “Given the relatively limited size of the training dataset, and the experience level of the human classifiers, this is a remarkable result.”
All this bodes well for the development of machine learning tools in the field of archaeology and anthropology — but it also begs the question: if machine learning can sort sherds, is it really that difficult for them to tell how many of these pictures contain a stoplight?
Related
Source: https://hyperallergic.com/651726/machine-learning-matches-ancient-pottery-fragments-tusayan-white-ware/