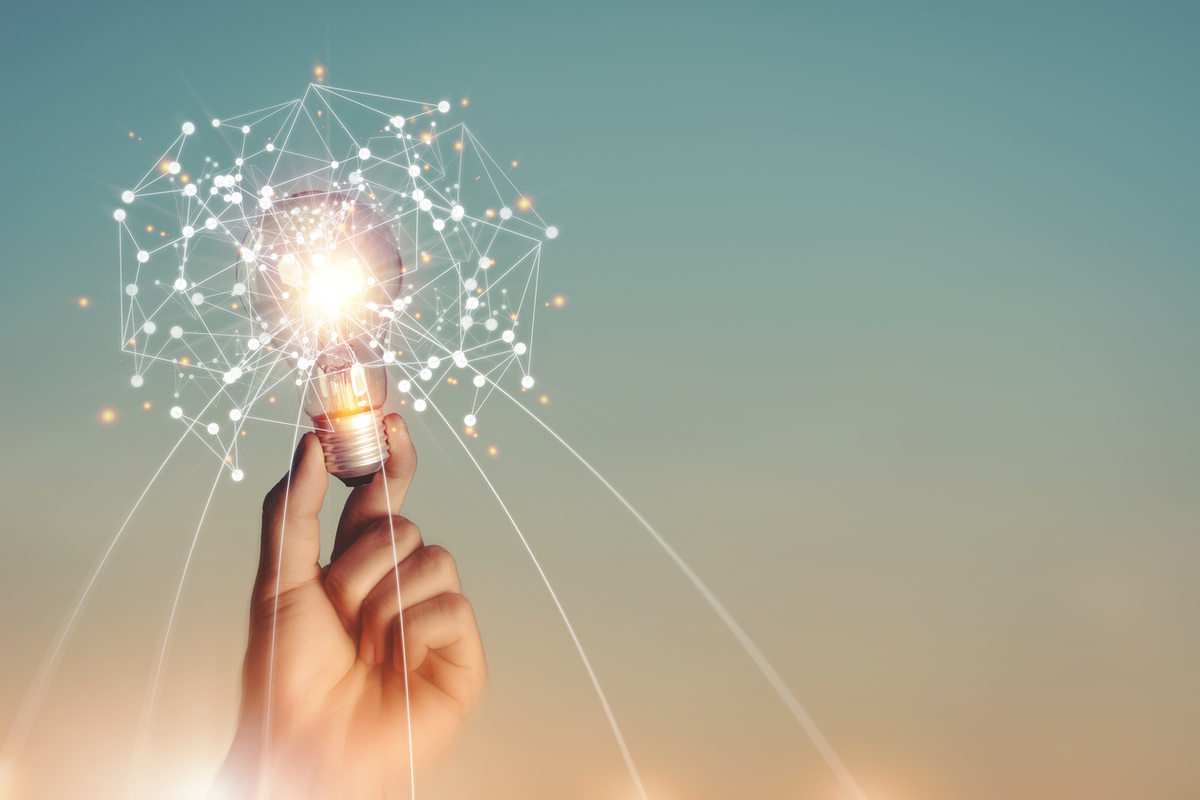
A recent Gartner report found that companies with AI experience were only able to move 53% of their proof-of-concept projects into production over the previous two years. Factors for the stalled projects or outright failures included a lack of machine learning (ML) skills, organizational inertia, and having the right quantity and quality of data for the models.
If you’re looking to re-invigorate a stalled ML project, it’s a good idea to take a step back and explore the reasons why you’re stuck.
The best place to start is with the data. While companies are generating a lot of data, they often struggle with how to manage it and get it prepared for ML. “You want to make sure you have enough clean data that is structured in the right way so you can use it to create machine learning models,” says Bratin Saha, Vice President and General Manager of Machine Learning Services at Amazon. “You may find you have the right amount of data, but you need a more sophisticated model. Usually, you can take what you’ve built and improve and iterate on it.”
Second, consider the support you’re getting for ML initiatives. Many organizations often don’t have executive buy-in from the start, or executives may lose interest after a few months if they’re not seeing tangible outcomes. For this reason, it’s critical to start with the right use case – and measurable outcomes – before beginning a project. “Machine learning is often an iterative process,” says Saha. “It takes a few months to train the model and make sure it is making the right predictions. You have to persevere in order to do this, which means finding the right use case.”
Teams also need to ensure they have access to the right tools including infrastructure – and the right skills. “If you have to build that infrastructure, you are taking effort and time away from doing the machine learning aspect of it,” says Saha. “Having access to the latest cloud technologies makes it much easier and more likely that you’re going to succeed.” Today, there aren’t enough people today who can build ML models, so closing the skills gap is also critical. In-house training is important as is adopting tools that make ML easier.
AWS offers several tools and services for organizations that don’t have deep in-house ML expertise. Amazon’s SageMaker JumpStart, for example, helps developers get started quickly with ML by offering a set of solutions for the most common use cases that can be deployed with a few clicks, including predictive maintenance, fraud detection, credit-risk prediction, churn prediction, and personalized recommendations, among others. The service provides one-click deployment and fine-tuning of more than 150 popular open-source models in areas such as natural language processing, object detection, and image classification models.
AWS also offers customers access to its Machine Learning Solutions Lab, where Amazon pairs its data scientists with customers to collaborate on defining and deploying a project, right through to a successful business outcome. Saha suggests that companies looking to jumpstart an ML project read through successful use cases and references with companies that found success through their projects. With more than 100,000 customers using AWS for ML, there are plenty of examples for companies that may be looking to revive an earlier project.
As with any IT project, proper planning goes a long way to delivering on an ML investment. “The more upfront planning you do in terms of defining the business problem you’re looking to solve, how it maps to the machine learning modeling and the data set you need, and the tools you’re going to use, the more you can increase your chance of success,” says Saha.
Learn more about ways to put your data to work and reinvent your business.
Source: https://www.cio.com/article/3621730/how-to-jump-start-machine-learning-production.html