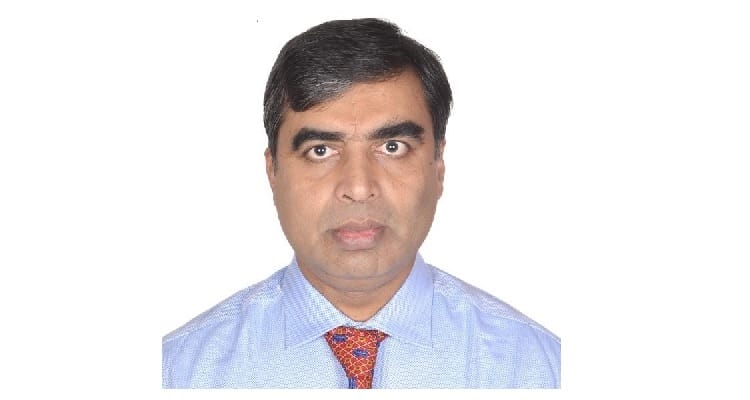
Read the article Satish Kumar, Suparna Systems CEO of Bangalore cites many of the benefits of AI and ML, allowing these systems to reduce the burden of management and documentation and focus more on patient care rather than replacing medical caregivers. Repeatedly states that it will be L The adoption of artificial intelligence (AI) / machine learning (ML) will disrupt the medical services provided by government or private hospitals and clinics. This improves patient care and satisfaction and reduces shipping costs. It also helps address the serious shortage of trained personnel in the medical field. This makes it easier to access medical services even in rural areas. Patients interact with the hospital for appointments, consultations, hospitalizations, laboratory diagnoses, discharges, and more. In recent years, several private and publicly funded hospitals have adopted digital tools to automate workflows and patient records. Hospitals have introduced electronic systems such as bookings, hospitalizations, diagnostics / tests, claims, insurance claims and pharmacies. The record link that connects all these records for a particular patient remains a concern. AI can enhance and enhance patient interactions and workflows from primary care to the emergency room. Useful for EMS and emergency medical services. The AI-enabled symptomatology checker can screen patients even before they visit a clinic or hospital. Chatbots can answer common questions about insurance eligibility, out-of-pocket costs, and help you book your booking. Screening chatbots can collect patient information and symptoms and identify the correct area of expertise in which a patient can be booked. This helps reduce waiting time in the hospital and bring patient satisfaction. John Hopkins Medical College has used deep learning (a sub-branch of AI) to improve ophthalmic critical care. A few years ago, researchers at Stanford University demonstrated that the ML model can better identify cardiac arrhythmias from the ECG than experts. ML can use electronic health records (EHR) to build predictive models. EHR is clinical data about a patient, with details such as medical history, diagnosis, laboratory test results, prescribed medications, and treatment plans. EHR datasets are complex and non-uniform. You can use boosting and logistic regression ML techniques to predict serious conditions such as heart disease. The ML model can be trained on previous EHR records that include serious illnesses such as heart failure that require prediction. To be able to use it in real life, the ML algorithm needs to be thoroughly trained individually for each disease or medical condition. This requires a huge amount of data for training and validation. This is fortunate to be available due to the widespread adoption of EHR. Model training is a costly and time-consuming process. The trained model must be approved by the drug regulator before it can actually be implemented. If the model needs to be used in different countries, it will require retraining and approval from country-specific regulators such as the US FDA and the European EMA. AI can also be used for risk stratification. Patients can be divided into high-risk and low-risk groups. In emergency room situations, it helps predict the likelihood of death or admission to the ICU. A study conducted to compare artificial neural network (ANN) -based predictive models with Acute Physiology and Chronic Health Evaluation II (Apache II) models to predict ICU mortality using the same physiological variables. I have a study. The performance of the ANN-based forecasting and classification model was consistent and found to be superior to APACHE II in several respects. For practical use, the model must be trusted not only by the doctor but also by the patient. Many healthcare providers are hesitant to adopt these models because they do not know how the diagnostic decision was made. These ML algorithms, especially deep learning algorithms, act as black boxes. This basically means that you get results when you run on the data. You do not know the logic and reasoning behind your results. This creates an unreliable situation for patients and doctors. However, much research has been done in recent years to make it transparent and explainable. In addition to clinical systems, AI / ML models also help reduce the administrative workload of healthcare providers. AI can be used to automatically generate prescriptions from patient-doctor interactions. Auto-generated prescriptions can be run through a physician for approval and can be incorporated into clinical workflows. These systems also help optimize hospital resources such as beds and medical devices. With the large number of high quality open source systems and libraries available, you can build your model in a cost-effective way. You can use TensorFlow and PyTorch for deep learning. You can use open source libraries such as AllenNLP and StanfordNLP for natural language processing. The more you use the model, the better it will be and the more accurate it will be. From the IT side, to implement them and keep them up to date, you need to build what is widely known as MLOps. Organizations need to build a pipeline of continuous delivery and automation. You can use open source workflow orchestration software such as Apache Airflow for this. Since these models use personally identifiable information (PII), you need to implement a way to address your privacy concerns. Security remains of paramount importance in these systems. The principle of defense in depth should be used to build a defense-in-depth architecture. These AI algorithms do not replace the work of the healthcare provider, reducing the burden of management and documentation and allowing more focus on patient care. One of the main reasons is that the algorithms do not understand the trade-offs, the patient care practice is an art, and most of the time the trade-offs are involved. Also, AI only gives probabilities, not certainty. AI can show optimal / relevant choices, and doctors can choose one of them. Healthcare transformation is certainly progressing with the adoption of these AI / ML models. (Views are personal)
Adoption of AI/ML can disrupt healthcare services Source link Adoption of AI/ML can disrupt healthcare services
Source: https://californianewstimes.com/adoption-of-ai-ml-can-disrupt-healthcare-services/390013/