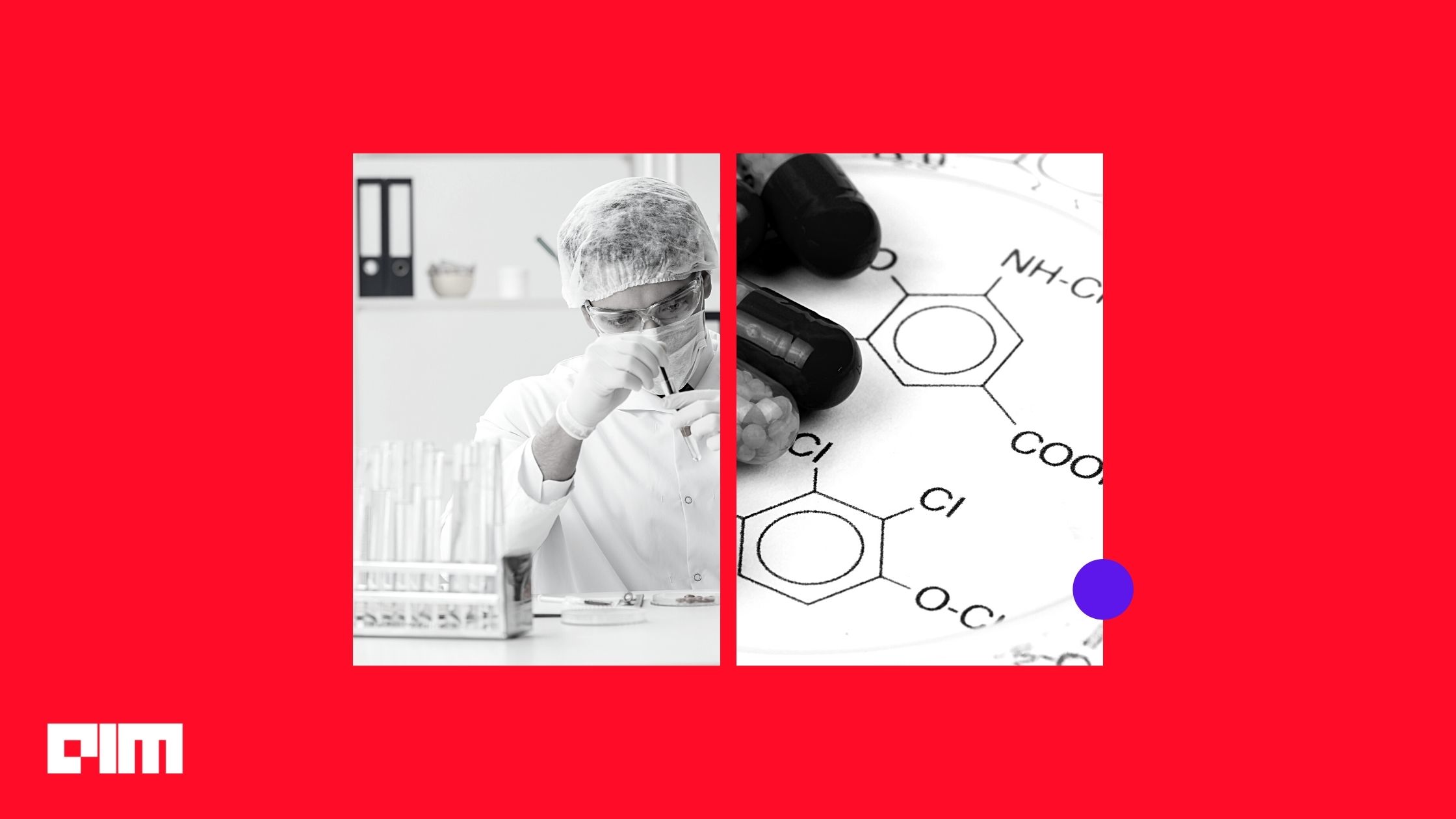
The University of Liverpool researchers have conducted a study to predict over 20,000 unknown associations between known viruses and susceptible mammalian species. The research, published in a paper titled. “Divide and conquer – machine-learning integrates mammalian, viral, and network traits to predict unknown virus-mammal associations”, used machine learning to improve our understanding of viral host ranges apropos mammals.
“Host range is an important predictor of whether a virus is zoonotic and therefore poses a risk to humans. Most recently, SARS-CoV-2 has been found to have a relatively broad host range which may have facilitated its spill-over to humans. However, our knowledge of the host range of most viruses remains limited,” said lead researcher Dr Maya Wardeh from the University’s Institute of Infection, Veterinary and Ecological Sciences.
Host range is key to whether a virus is zoonotic ( if it can be transferred from animals to humans) and poses a threat to humans. For For example, SARS-CoV-2 has a broad host range, including bats, cats, ferrets etc. Interestingly, less than 1% of mammalian viral diversity has been discovered so far. However, we can leverage the current knowledge to gauge the extent to which we are under-observing associations between known viral agents and their mammalian hosts. The topological features of the network have been vetted to gain insights into patterns of pathogen sharing, disease emergence and spill-over events and also to predict missing links in a litany of host-pathogen networks.
Machine learning framework
The researchers incorporated the global view of viral sharing into a machine-learning driven framework to predict unknown associations between known viruses and their mammalian hosts. The framework took a multi-perspective approach to predict the viral mammal associations thrice from the vantage points of mammal, virus and the network. The results were consolidated via majority voting.
The researchers transformed the species-level virus-mammal associations into a bipartite network in which nodes represent either virus or mammal species, and links implied associations between mammalian and viral species.
The framework trained and selected a set of supervised classifiers in each of the three perspectives. Then it consolidated the results of the best performing classifiers using voting whereby an unknown association was selected if it was predicted by at least two of the three perspectives.
From the mammalian perspective, the probability of a virus affecting/associating with this focal mammal was quantified based on our knowledge of the viruses found in this mammal. From the viral perspective, the probability of the virus infecting/associating with included mammalian species is quantified based on our understanding of known hosts of this virus. The final perspective enables predictions based on the topology of the network linking all included mammals with all included viruses.
The framework is flexible, in terms of machine-learning algorithms selected, classifiers trained, and features engineered for each perspective. It avoids overfitting as it approaches the problem from various perspectives, and effectively consolidates ensembles of classifiers trained on subsets of the underlying data. Moreover, no constituent model of the framework has been trained with all available data at any time. The framework also enables the incorporation of hosts where only one virus has been detected to date (via perspectives 2 and 3), and viruses where only one host has been discovered (via perspectives 1 and 3).
Wrapping up
The research predicted a 5.35-fold increase in associations between wild and semi-domesticated mammalian hosts and known zoonotic viruses. The results pointed to a 5.20-fold increase between wild and semi-domesticated mammals and viruses of economically important domestic species. Not surprisingly, bats and rodents are linked with increased risk of zoonotic viruses.
“As viruses continue to move across the globe, our model provides a powerful way to assess potential hosts they have yet to encounter. Having this foresight could help to identify and mitigate zoonotic and animal-disease risks, such as spill-over from animal reservoirs into human populations,” said Dr Wardeh.
#wpdevar_comment_1 span,#wpdevar_comment_1 iframe{width:100% !important;} #wpdevar_comment_1 iframe{max-height: 100% !important;}
Join Our Telegram Group. Be part of an engaging online community. Join Here.
Subscribe to our Newsletter
Get the latest updates and relevant offers by sharing your email.
Source: https://analyticsindiamag.com/how-machine-learning-predicts-the-host-range-of-known-viruses/