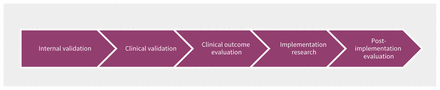
KEY POINTS
Evaluation of machine-learned systems is a multifaceted process that encompasses internal validation, clinical validation, clinical outcomes evaluation, implementation research and postimplementation evaluation.
Approaches to clinical validation include comparisons of model performance with those of clinician experts and silent deployment of systems with comparisons of predictions to actual patient outcomes; clinical outcome evaluation can be done through randomized controlled trials, cohort studies, interrupted time series analyses and before-and-after studies.
Implementation research includes qualitative and quantitative components and formative assessments and is attentive to the context in which the system is being deployed while evaluation frameworks can help teams structure their studies and analyses.
Postimplementation evaluation is necessary to monitor for and account for threats to system performance after deployment, which may necessitate retraining and recalibration of machine-learned systems.
A multidisciplinary team comprising data scientists, clinician experts and implementation scientists (qualitative and quantitative expertise) can help ensure that a comprehensive evaluation is undertaken before, during and after deployment.
Related articles have outlined problems with the development of machine-learned solutions for health care and suggested a framework for their optimal development.1,2 The spectrum of clinical settings in which machine learning approaches have been examined for use in the health care setting has increased markedly and become more diverse in recent years. Many studies have detailed the data science and statistical bases of machine-learned tools.2 However, comparatively few studies have focused on their evaluation and implementation.3 We discuss how to evaluate machine-learned solutions throughout their life cycle to optimize their use and functionality in clinical practice. Internal validation — that is, ascertaining the discriminative and calibration performance of an algorithm — should be followed by evaluation of both performance and outcomes of interest in the clinical setting, as well as evaluation of the tool’s implementation into existing workflows (as outlined in Figure 1).
Evaluation life cycle of machine-learned systems in health care.” data-icon-position data-hide-link-title=”0″>
Figure 1: Evaluation life cycle of machine-learned systems in health care.
What is the process of model or algorithm development and interval validation?
Initially, evaluation of the predictive performance of machine-learned algorithms involves assessing their discriminatory and calibration accuracy. The former quantifies the ability of the algorithm to separate individuals according to the presence or absence of a given outcome, and the latter measures how close the predicted probabilities are to actual probabilities.4 Such experiments comprise the internal validation stage of machine-learned algorithm development and represent the majority of published reports describing machine learning in medicine.3
Typically, studies determining the predictive performance and accuracy of different algorithms are retrospective in nature. Large, historically labelled data sets are used to train and test algorithms.3,5 Machine learning methods employed at this stage range from relatively familiar approaches such as linear or logistic regression to more complex neural networks and natural language processing models.5,6 In all cases, algorithms are first “trained” on the largest portion of the data reserved for this purpose, and then evaluated on the remaining data, referred to as the test data.3–5 When the outcome of interest is binary (e.g., disease present or absent), performance is typically reported using standard measures such as sensitivity, specificity and the area under the receiver operator characteristic curve.5,7 For continuous outcomes (e.g., predicted dose of a medication), performance is generally quantified using measures such as the root mean squared error or mean absolute error.8 Graphical methods, such as calibration slopes and calibration curves, can be used to assess model calibration.9
Although the need for clinician or stakeholder input at this technical stage of development may not be immediately apparent, clinicians can provide important insights regarding the interpretability of performance metrics and acceptable thresholds of model performance for clinical practice.10 For example, as part of the development of a machine-learned-based early warning system predicting patient deterioration and need for intensive care within a 24-hour period, a maximum of 2 false alarms per true alarm was identified by clinicians as an acceptable threshold for performance to guard against “alert fatigue.”1 Based on this requirement, it was determined that the system should have a positive predictive value of at least 0.3 while detecting as many deteriorating patients as possible.1 Because optimal performance metrics will vary by clinical context, defining performance will therefore require consideration of clinician preferences and the care environment in which the machine-learned system will ultimately be operating.1,10
How should machine-learned solutions be validated clinically?
Performance of machine-learned tools on real-world data that are new to the algorithm may differ from performance during internal validation.2 Consequently, prospective studies that compare predictions made by machine-learned algorithms with clinician predictions are required to ascertain their performance in a clinical setting. As described in our related paper, this approach was used as part of the evaluation of a machine-learned early warning system for patients on medical wards designed to identify who may require critical care; in this evaluation, we found improved sensitivity of the early warning system over prediction by clinicians.1 Other examples include comparisons between machine-learned systems and dermatologists for diagnosing skin cancers;11–14 diagnosis of age-related macular degeneration and diabetic retinopathy using retinal optical coherence tomography or fundus photographs;15–17 identification of breast cancer metastases in lymph node biopsies;18,19 and detection of polyps at colonoscopy.20,21
Another approach to clinical validation involves comparing the performance of a newly developed machine-learned algorithm against already validated clinical risk–scoring tools that are commonly used in clinical practice. This approach has been applied to various problems; e.g., predicting gastrointestinal bleeding and mortality after cardiac surgery.22,23 As with approaches involving predictions by clinicians, comparisons with validated risk-scoring tools should be undertaken using data that were not part of the machine-learned model’s development process.
Although many studies have shown the performance of machine-learned tools to be at least comparable to the performance of expert physicians, this is not always the case,24 which underscores the need to conduct clinical verification studies before moving forward with more resource-intensive forms of evaluation. Clinical validation can be particularly challenging when diagnostic interrater reliability among clinicians is poor. In this context, it may be difficult to compare the discriminative performance of clinicians versus machine-learned systems, given the challenges associated with discriminating between the presence or absence of disease or associated stages of illness (e.g., remission, relapse). Potential strategies for addressing this problem include use of more concrete, measurable aspects of a specific illness (e.g., change in symptom scores or laboratory parameters) or a directly observable functional outcome (e.g., ability to return to work) rather than diagnostic labels denoting the presence or absence of disease when training models.
“Silent deployment” is another approach that may be used for clinical validation. As described in a related article, the machine-learned system runs in a silent mode and generates predictions, yet these are not communicated to clinicians and therefore do not influence care.1 Although silent deployment typically focuses on issues related to technical deployment and workflow and does not involve clinical interventions, predictions made by the tool during silent deployment can be compared with the actual patient outcomes, which allows for estimation of the algorithm performance.
Large data sets are generally not required for the prospective validation of machine-learned algorithms. Instead, sample sizes can be estimated using established methods for studies of test accuracy.25
How can we establish whether machine-learned solutions improve patient outcomes?
Establishing and verifying predictive performance through internal and clinical validation studies does not answer the fundamental question of whether patients benefit from the integration of machine-learned solutions into clinical practice.26 Generating robust evidence that supports the impact of such algorithms on patient outcomes is a prerequisite to widespread implementation in clinical practice and investment in resources and infrastructure required to continuously monitor the performance of such tools once deployed is needed.
As with other interventions, randomized controlled trials (RCTs) are the gold standard for establishing the efficacy of interventions developed through machine learning. Yet, relatively few RCTs of machine-learned interventions have been registered or published.3,27 These include a double-blind RCT of an algorithm to detect acute neurologic events and a trial comparing automated interpretation of cardiotocographs with usual care on clinical outcomes in mothers and infants.28,29 Possible reasons for the dearth of RCTs in the field of machine learning include the need for large samples of patients or long durations of follow-up to show efficacy, cost and concerns regarding intervention fidelity or cross-group contamination when trials are conducted within the same institution. Although cluster RCTs could address the latter issue, these studies add to the logistical and methodological complexities inherent in multisite trials.30,31
Because conducting RCTs is challenging, other approaches are often used for generating evidence of clinical benefit of machine-learned systems, such as matched cohorts, quasiexperimental interrupted time series analyses, and prospective before-and-after studies.32–34 In a related article, we described how we planned to use an observational matched cohort study design to evaluate a machine-learned early warning system in a General Internal Medicine unit, given that an RCT was estimated to require about 25 000 patients.1 Although findings from observational studies are often considered to be a lower level of evidence than RCT findings, they provide a compromise between the needs of stakeholders and clinicians seeking timely evidence of clinical impact with machine-learned interventions and the resources required to conduct RCTs.
How can the implementation of machine-learned solutions be optimized?
Despite the potential of interventions developed using machine learning to assist with clinical decision-making and improve clinical workflow, only a few examples of successful deployment in clinical practice currently exist.35 Moreover, studies that describe the steps taken to translate machine-learned algorithms into clinical tools are few. However, such studies are important for identifying and addressing social, ethical, organizational and logistical barriers to adoption. Implementation science — the study of methods for promoting the uptake of interventions into routine practice — should therefore be considered as fundamental as data science and clinical outcome evaluation for integration of machine-learned systems into clinical practice.36,37 Although a detailed exposition of implementation science is beyond the scope of this article, several points merit emphasis.
In contrast to internal validation and clinical research, which emphasize the performance and efficacy or effectiveness of machine-learned solutions, implementation science research questions and outcomes focus on the process of implementation, and could include measures of intervention uptake or acceptability; they may characterize provider perceptions of the intervention on established workflows, as well as changes in processes of care.37 In addition, understanding the context in which the machine-learned system is being implemented is important for optimizing uptake.36 This requires addressing questions such as how to best align the system with existing workflows, how to customize the end-user interface in a manner that minimizes disruption to existing practices and which members of the care team will be interacting with the system.
Quantitative and qualitative approaches can be used for implementation research. Quantitative data can be derived through the use of structured surveys, administrative health databases, electronic health records and decision support systems, depending on the outcomes being examined.38 Surveys can be used to ascertain facilitators and barriers to implementation, attitudes about the integration of the system in established workflows and acceptability of the intervention. Health records can be sources of information regarding intervention uptake, quality of care and costs. Qualitative methods can add depth and contextualization to quantitative approaches by examining how and why an intervention is or is not being used by clinicians, providing potential insights into interprofessional or organizational dynamics that influence uptake, and sociocultural barriers to implementation.39 Qualitative data may be generated through in-depth interviews, focus groups, document analysis or observation, depending on the research question(s) and methodologic or theoretical orientation of the researcher.
Formative evaluations, wherein data are generated and shared with the research team and target clinicians at different stages of implementation, allow an implementation team to troubleshoot challenges arising during implementation and adapt the solution to better integrate into care processes.40 Using an evaluation framework or theory when studying the implementation of machine-learned tools can assist researchers in structuring their studies and specifying concepts that warrant measurement. Readers are referred elsewhere for an overview of commonly used evaluation frameworks in implementation research.41
Why is ongoing postimplementation evaluation necessary?
Because clinical practice and processes evolve over time, the evaluation of machine-learned solutions does not end with implementation. Instead, ongoing evaluation of such systems is required to continuously monitor performance. An important threat to their performance is data-set shift, where temporal changes in clinical practice or the distribution of patient characteristics result in a data set that differs from that which was originally used to train the algorithm.42–44 This can occur, for example, if a machine-learned algorithm is used to make clinical predictions on data from an increasingly ethnically diverse population, or a new site with a different patient population from the training data set.2,45 Other data-related threats to system performance could include changes in the variables that were originally used in model training, such as the addition of new categories or an increasing frequency of missingness in selected variables.
Evaluating ongoing system performance may incorporate several steps,46–49 including regularly retraining systems with the most recent data sets, comparing model performance on updated data with data currently in use and investigating discrepancies; updating outcome definitions and model inputs to align with evolving disease epidemiology, treatment or pathophysiology; generating alerts that are triggered when variable frequency distributions change; and regularly consulting with clinical experts to monitor changes in system performance and ensure sustained clinical relevance. Where feasible, post-implementation evaluation of machine-learned solutions should be automated and scheduled at regular intervals to detect, investigate and resolve sources of system deterioration expeditiously.
Conclusion
Evaluation of machine-learned solutions is a multifaceted process that requires the expertise of data scientists, clinician experts and implementation scientists. Presently, most literature describing evaluation of these solutions remains focused on internal validation, with relatively few studies examining clinical outcomes and system implementation. This imbalance has contributed to what has been referred to as the “artificial intelligence chasm,” representing the gap between the development and validation of machine-learned algorithms and their eventual use in clinical practice.43 Additional clinical outcomes and implementation research is therefore necessary to fully realize the potential of machine learning in medicine.
Footnotes
CMAJ Podcasts: author interview at www.cmaj.ca/lookup/doi/10.1503/cmaj.210036/tab-related-content
Competing interests: None declared.
This article has been peer reviewed.
Contributors: Both authors contributed to the conception and design of the work. Tony Antoniou drafted the manuscript. Muhammad Mamdani revised it critically for important intellectual content. Both authors gave final approval of the version to be published and agreed to be accountable for all aspects of the work.
This is an Open Access article distributed in accordance with the terms of the Creative Commons Attribution (CC BY-NC-ND 4.0) licence, which permits use, distribution and reproduction in any medium, provided that the original publication is properly cited, the use is noncommercial (i.e., research or educational use), and no modifications or adaptations are made. See: https://creativecommons.org/licenses/by-nc-nd/4.0/
Source: https://www.cmaj.ca/content/193/36/E1425