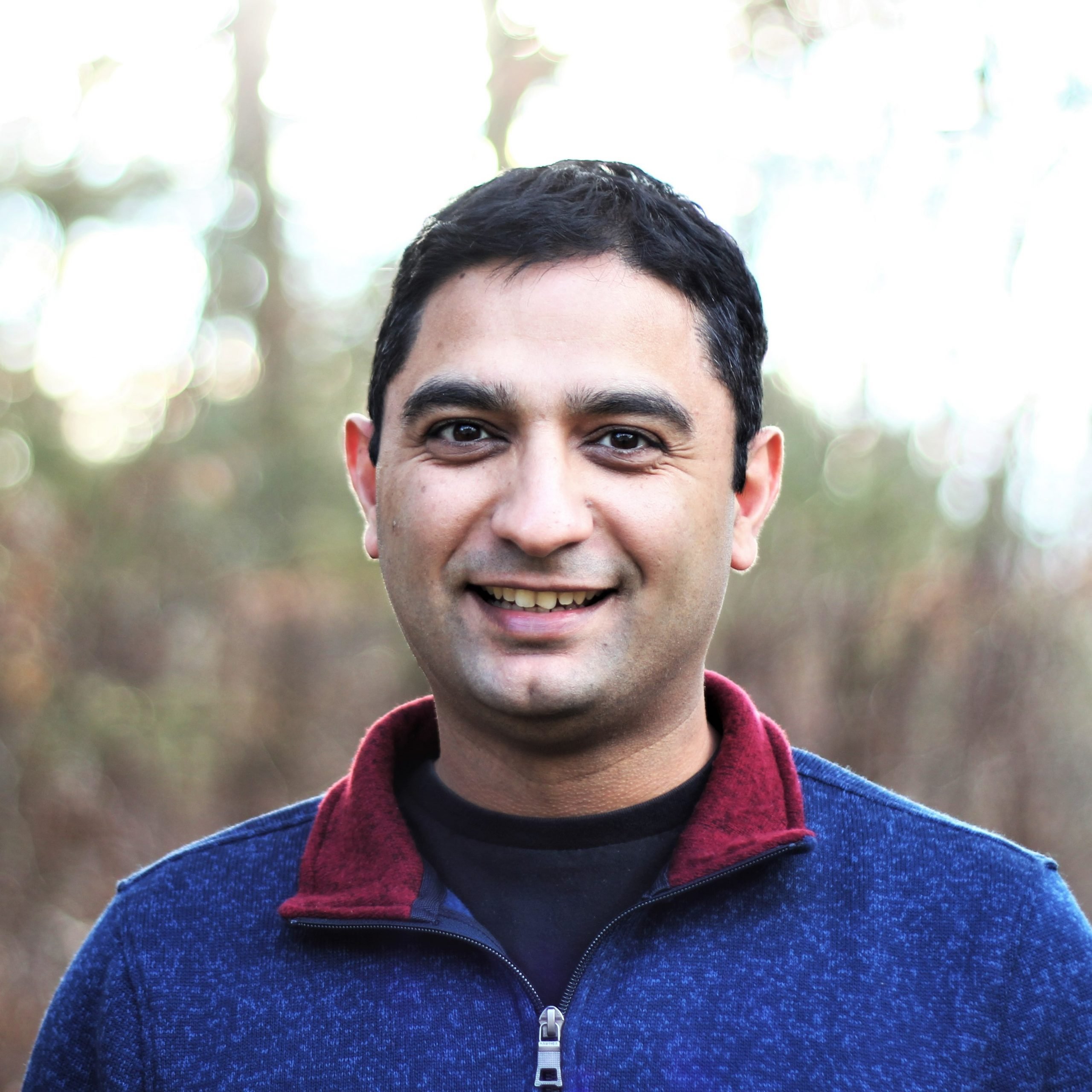
Dynatrace sponsored this post.
Even before the COVID-19 pandemic, IT operations were under increasing pressure.
Bipin Singh
Bipin is director of product marketing — platform and AIOps at Dynatrace. He is a 15-year veteran in software and infrastructure for data management, machine learning and AI. He has held roles in marketing, product management and engineering at TIBCO, Nexla, Carl Zeiss and Intel. Bipin holds an MBA from Babson College, a Ph.D. from Iowa State University and a bachelor’s degree in chemical engineering from the Indian Institute of Technology Kanpur.
Companies racing to usher in digital transformation forced enormous change onto developers. Organizations generated data at a jaw-dropping pace. With its dizzying combinations of multicloud and hybrid cloud strategies, cloud computing heaped even more complexity on IT operations. Therefore, organizations need to place artificial intelligence (AI) at the center of enterprise software’s next cycle.
Today, as organizations accelerate digitalization efforts, more have turned to AI-powered software intelligence to enable greater intelligent automation and vertical integration.
Companies must boost efficiency and simplify processes as they migrate to multicloud architectures and embrace microservices, containers and other cloud native technologies. When it comes to managing complex modern cloud environments, machine-learning-based approaches must give way to true AIOps systems and practices.
Basic Machine-Learning Tools Demand too Much from Humans
Consider the business environment: One fault can affect countless connected services. Additionally, distinguishing between normal and faulty application behavior is challenging.
As it stands, traditional monitoring tools rely on machine learning — a statistical solution — to uncover the source of problems. To identify faults, machine-learning-based AI correlates events, application-performance metrics and alerts.
These solutions need to be trained. And because a single fault can trigger an alert storm, the warning bells aren’t that helpful. Additionally, machine-learning tools too often fail to identify unknowns and an issue’s root cause. Just as critical, most traditional methods play a small role in fixing issues.
Therefore, making sense of alerts and tracing them back to the root cause — an often arduous and time-consuming job — typically falls to humans.
AIOps Supplies the Answer and Automation
In contrast, deterministic AI scours every crack and crevice of a stack in real time for every relevant piece of data, allowing it to build an accurate fault-tree analysis. Deterministic AI generates a topological relationship map that enables visualization of affected components and understanding of how everything links together. Because the AI has all the data from every component of the stack and knows how different entities are related, it can identify the root cause with speed and precision.
That’s when the best AIOps platforms can initiate auto-remediation procedures, even before most users are aware of glitches.
Ultimately, machine learning vs. AIOps boils down to this: Software driven by rudimentary machine learning can only make educated guesses about the cause of faults and performance issues while depending on humans to make the call. Deterministic AI tools, on the other hand, correctly identify faults and equip IT operations with precise answers rapidly. AI then enables automatic and pain-free problem-solving. This slashes the amount of time spent hassling with triage and research.
The topology map and problem-evolution data are critical to the auto-remediation process. The remediation process can be triggered via application programming interfaces, or APIs, to precisely resolve problems at a speed humans can’t match.
Another key component to creating a self-healing system is an observability platform that offers end-to-end visibility. The need for this kind of observability is extensive. Holistic observability platforms provide answers and visibility from user experience, applications and infrastructure through a seamlessly connected intelligence with AI at the core. With only 5% of applications monitored, there is a significant opportunity for organizations to modernize their monitoring approach.
AIOps Grows Organically within Organizations
To this point, the migration from machine-learning-based observability to AIOps observability occurs mostly organically. We’ve seen how a single team — one that might struggle to meet service-level objectives — begins to look for ways to become more efficient.
The team might spend hours each day maintaining IT infrastructure and resetting or restarting systems. But this manual approach prevents them from properly maintaining their systems overall.
Typically, other business units then recognize the opportunity to automate their old manual processes as well. AIOps-enabled observability can provide time- and cost-saving automation. It enables teams to go from reactive to proactive.
By adopting automated incident remediation or closed-loop remediation, the team doesn’t have to wait for a problem to crop up to act. When a fault occurs that crosses the threshold, the team has proactively configured the system to automatically launch intelligent solutions for fault correction, thus creating a self-healing system.
The many advantages of AIOps-enabled observability include the bridge it creates between site reliability engineering, DevOps and IT operations teams. These teams rely on disconnected dashboards, and a single observability platform enables each to draw information from a single source of truth.
Creating More Dashboards Is Not the Goal
Given the current overdependence on dashboards, it’s time to change our thinking about visualization. Certainly, dashboards are important for understanding data. But for so many tools today, the end output is a dashboard that still needs human expertise to make sense of it.
Organizations are tired of getting stuck with a fancy dashboard that slices and dices data in different ways but produces only data outputs. Someone must still interpret that data to take action. Teams want their tools to go further and carry more weight.
As organizations move into the post-pandemic era and as the security climate remains fraught with threats, more IT leaders will shift to intelligent, automated and self-healing systems.
Feature image via Pixabay.
Source: https://thenewstack.io/machine-learning-vs-aiops-battle-for-better-answers-and-automation/