zurück zum Artikel
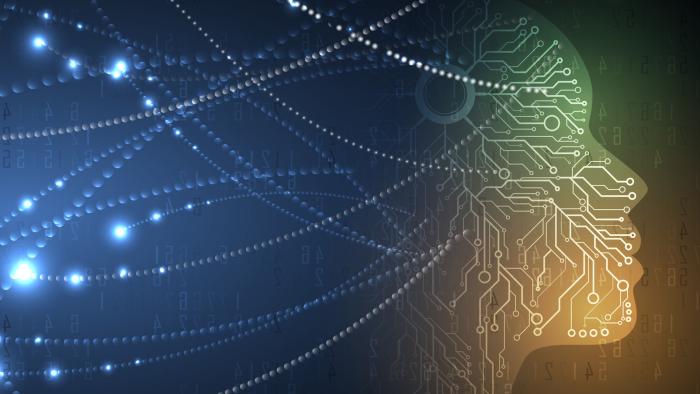
(Bild: vs148/Shutterstock.com)
Artificial intelligences are becoming more and more common these days. In our FAQ, we clarify the most important questions about AIs.
(Hier finden Sie die deutsche Version des Beitrags [1])
Artificial intelligence (AI) has become one of the biggest hype topics in computer technology over the past few years. And not without good reason: from voice assistants to self-driving cars to use in industry, AIs are already being used in many areas of life – and new promising applications are set to follow in the next few years. But which of these promises are actually really good ideas and which are not? What can artificial intelligence do already and what not? And what is really true about the horror dystopias about all-powerful superintelligences? Together with our colleague Pina Merkert, editor at c’t, we explain the most important basic terms and questions about the field of artificial intelligence.
Artificial intelligence, machine learning and neural networks – what is that supposed to be?
An artificial intelligence (AI) is an algorithm that can imitate functions otherwise attributed to a human brain. For example, it is capable of learning, can independently extract patterns from data and solve problems based on them. For example, an AI can learn to distinguish between dogs and cats and then recognise the animals in pictures.
The field of artificial intelligences contains a large number of algorithms of different types. Probably the best-known type of AIs are the so-called machine learning algorithms. As the name suggests, these algorithms can learn and adapt themselves to new data. This means they are no longer dependent on developers who tell them step by step how to solve a problem.
The term machine learning in turn covers a number of different algorithms with diverse use cases. For example, support vector machines or K-nearest neighbour algorithms should be mentioned here. The top dogs among machine learning algorithms, however, are neural networks. While other machine learning algorithms are difficult to scale up, neural networks can be made larger and more complex.
A neural network can be thought of as a large number of small computers interconnected like the network of synapses in the human brain. On the data presented to the network, it applies pattern recognition at different levels. If the training works as it should, the patterns become more abstract and useful for making decisions or predictions from layer to layer. As with the brain’s synapses, training can strengthen connections between individual neurons in the neural network and weaken other connections. The network thus learns that certain connections are important and encodes that in its parameters. With each additional data point, the AI thus becomes more and more precise; the AI is “trained”.
What is the difference between strong and weak AIs?
All of today’s artificial intelligences fit under the heading of weak AI. They are often only intended to solve a specific problem; they cannot perform tasks outside their area of responsibility. They are thus quite limited in their scope of application. Nevertheless, the algorithms have two major advantages: On the one hand, some AIs are significantly more precise than humans in their specific problem field, others are faster and all are cheaper. Secondly, developers do not have to programme the problem solution by hand. Instead, they train an AI with a data set so that the result is just as good as if it was created by a human.
A strong AI, on the other hand, would be an algorithm that behaves like a human being as a whole. The AI is then no longer limited to application to a specific problem, but can perform all tasks that a human can perform – and at least as well or significantly better. This form of AI does not yet exist in reality. Although research is pursuing the goal of a strong AI, it is currently at least years, probably decades away.
How long has artificial intelligence existed?
The first beginnings in the field of AI were already made in the middle of the 20th century, although the algorithms at that time were significantly less large and complex than today’s algorithms. Even at that time, it was assumed that artificial intelligences could form a promising field of computer science. However, due to difficulties in optimising the algorithms, there was an “AI winter” around the 1970s, during which comparatively little money was invested in research. It was not until the 1980s that the field of artificial intelligences was researched more again due to the invention of so-called backpropagation. Since then, the research field has developed enormously fast.
Where are AIs already being used? And where can they still be used in the future?
Artificial intelligences are already used in many areas of life today. Private individuals come into direct contact with some of them, for example with image recognisers that can automatically search and sort photos, chat bots or speech models in voice assistants.
In many cases, however, consumers do not notice much about the use of AIs: For example, when a company uses AIs to determine the creditworthiness of people, or when artificial intelligence in industry detects faulty products and outliers in systems.
In the next few years, the biggest innovation in artificial intelligence that will affect private individuals is likely to be self-driving cars. Driving cars is a demanding challenge for AIs, as they must be able to steer the vehicles and react to obstacles and dangers in real time.
What can’t artificial intelligence do (yet)?
The biggest weakness of AIs is that algorithms do not think like humans. AIs rely on automated statistics to recognise patterns in data and act based on them. In many cases, this can work as well or even better than a human doing the same task. Sometimes, however, this kind of “thinking” also brings disadvantages.
This can be easily shown with an example: An AI was trained to identify dog breeds. The developers then checked how the AI went about distinguishing between the different breeds and discovered that it only counted the white pixels in a picture of a husky – because huskies are usually photographed in the snow and it was easier for the AI to analyse the environment for snow than to recognise the shape of a sled dog. A human being would not make such a mistake.
This is harmless when it comes to recognising dog breeds. However, it becomes more critical when, for example, a self-driving car is supposed to recognise obstacles. If the car detects people or objects incorrectly or not at all, serious accidents can occur.
Humans also have an advantage if you describe an object to them and ask them to imagine it. While we do not have much trouble filling in incomplete descriptions in our imagination, artificial intelligences still have a hard time doing this. They can sometimes generate photorealistic images based on the descriptions. However, when asked to represent parts of the object that were not included in the training data, they often produce bizarre results.
Will professions be replaced by artificial intelligence in the future?
As with every industrial shift, it would be quite naïve to assume that artificial intelligences will not eliminate jobs in the future. However, our colleague Pina Merkert estimates the danger of large numbers of full-time jobs being replaced by AIs as rather low. She considers it much more likely that simple, repetitive parts of a job will be taken over by AIs in the future, while humans will continue to perform the more demanding tasks. At the same time, AIs are likely to create new, highly skilled jobs. Humans will have to programme, monitor and maintain the algorithms.
However, AIs are mainly likely to take on tasks that are currently not being handled at all. For example, this could concern the moderation of comments on large websites: While today it is difficult to manually review all the comments published on a website, in the future an AI could check all comments for insults or inappropriate remarks.
What are the risks of artificial intelligence?
In Hollywood films, AIs are often seen as evil superintelligences that want to wipe out humanity. In fact, some researchers fear such a development: they speak of a singularity. This is a powerful AI that has become (significantly) more intelligent than humans through countless steps of self-improvement.
According to our colleague Pina Merkert, a singularity will probably remain science fiction for the near future. However, in her view, even today’s AIs bring dangers with them – especially if they are used incorrectly.
For example, a bias in the training data of an AI could affect the way it works. For example, if an AI is supposed to pre-sort job applications, it would make sense to feed it with training data from previous application processes – because the people who have already been hired should actually have been the most qualified. In reality, however, this may not necessarily have been the case: The decision for or against applicants could also have been influenced by discrimination, for example on the basis of gender or skin colour. These biases will then also be reflected in the decisions of the AI. In this way, a sexist or racist AI is created by mistake, even if this is not at all desired by the company.
Another disadvantage is that even the developers of an artificial intelligence can only understand to a limited extent how the AI came to a result. If a company uses an AI for decision-making processes, it may not be able to communicate exactly why a decision was made and how.
Furthermore, artificial intelligences – like any technology – can also be misused for malicious purposes. For example, AIs that are supposed to scan comment columns for insults or incitement could be used in a surveillance state to detect and delete government criticism.
Finally, our colleague points out that any technology that is relied on but does not function properly can be dangerous. Self-driving cars are another example of this: the AI that controls the vehicle should have collected enough training data to be able to deal with all dangers of road traffic. However, this is difficult in exceptional situations such as accidents, because it is difficult to collect training data from them.
URL dieses Artikels:
https://www.heise.de/-7239321
Links in diesem Artikel:
[1] https://www.heise.de/ratgeber/Kuenstliche-Intelligenz-Was-steckt-hinter-dem-Hype-7192364.html
[2] mailto:[email protected]
Copyright © 2022 Heise Medien