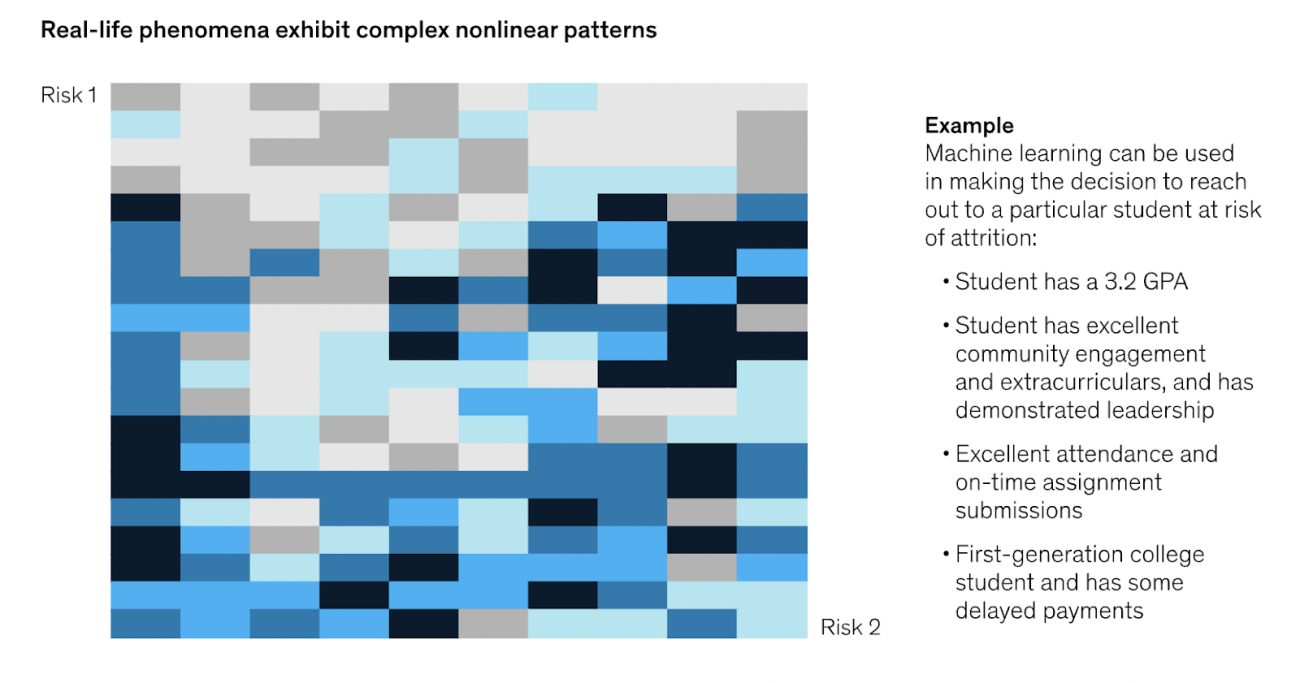
Listen to this story
September 8 has been celebrated as the ‘International Literacy Day’ across the world since 1967. The significance of this day arises from the fact that despite the steady rise in literacy rates over the past 50 years, there are still 773 million illiterate adults around the world. In India, though the literacy rate has seen phenomenal growth—from 18.3% to 74.4% between 1951 and 2018—there are 313 million illiterate people, according to the study, “Literacy in India: The gender and age dimension.”
Illiteracy and dropout rates are acutely linked. Dropping out of school is a rampant trend in India. As per the Economic Survey 2021-2022, the secondary level school dropout rate is 16.07% in 2019–20. However, early literacy intervention strategies could help arrest dropouts.
AI and ML at work in arresting dropouts
With the progress of technologies like AI, ML, IoT and data analytics, many higher education institutions are using these technologies as a part of their processes. The goal is to better identify the pain points in their students’ journey and efficiently allocate resources in the form of programme personalisation and flexibility so as to improve the overall experience of the students.
Institutions are even harnessing these technologies to identify students at risk of dropping out and reaching out to them proactively with personalised solutions. For example, by using predictive modelling, Western Governors University was able to increase the graduation rate of students enrolled in its four-year undergraduate programme by five percentage points between 2018 and 2020.
While ensuring that students enrolled in higher education do not drop out, it is also important that dropout rates at the school education level are arrested. Else, it can have serious repercussions on the overall literacy rate in the country as well as cognitive skill development. When students drop out of school, both students and communities lose out on these skills, talents and innovations.
In India, the Government of Andhra Pradesh, in association with Microsoft, used Azure’s machine learning platform to address the issue of school dropouts.
Using the ML platform, an application was developed that enabled the state education department to predict school dropouts. The application processed several data sets related to enrolment, student performance, gender, socio-economic demographics, school infrastructure among others to find predictive patterns for potential school dropouts.
Using this model, the Andhra Pradesh state education department was able to identify 19,500 probable dropouts in the Visakhapatnam district for the academic year 2018–19. The ML platform also provided an analysis of the key factors responsible for dropouts. Based on these inputs, the government initiated appropriate drives to increase enrolment at schools. They put in place awareness campaigns to enlighten pupils and parents about the importance of schooling.
Over the years, machine learning models have become the focus in addressing the problem of school dropouts. Researchers have made use of several advanced machine learning algorithms like logistic regression, decision trees and K-nearest neighbours, Multi Layer Perceptron and Deep Neural Networks to predict if a student will drop out or continue her education. In many recent studies, researchers have used deep learning to not only predict dropout rates but also provide personalised intervention to at-risk students.
ML algorithms could help identify students who are at risk of dropping out due to several indirect factors that may not be apparently deciphered using a linear rule-based approach. For instance, the case of students who are performing well in academics and have good attendance records but facing difficulty in paying the fees. While a linear rule-based approach may fail to identify such students at risk of dropping out, machine learning can help institutions identify such students beforehand and enable them to make direct appropriate interventions.
Despite the potential that machine learning holds in solving the problem of dropouts, not much research has been undertaken in this regard—especially in developing nations. For countries like India, there may be an urgent need to focus on research initiatives to come up with more robust and comprehensive early warning systems and identify students who are at risk of dropping out along with ranking students according to their probability of dropping. Such systems would allow institutions to take appropriate action and make necessary interventions.
The success of machine learning in arresting school dropouts would depend on availability of quality data. Timely and accurate data is a must for effective planning and decision making. In recognition of the importance of such data, the Ministry of Education through the Department of School Education and Literacy has come up with the online system of UDISE+, an improved and upgraded version of Unified District Information System for Education. This system is responsible for collecting real-time data on elementary and secondary education. The collected data can be used as inputs for ML algorithms to identify and mitigate the risks of dropouts.
Source: https://analyticsindiamag.com/machine-learning-to-deter-students-from-dropping-out-of-school/