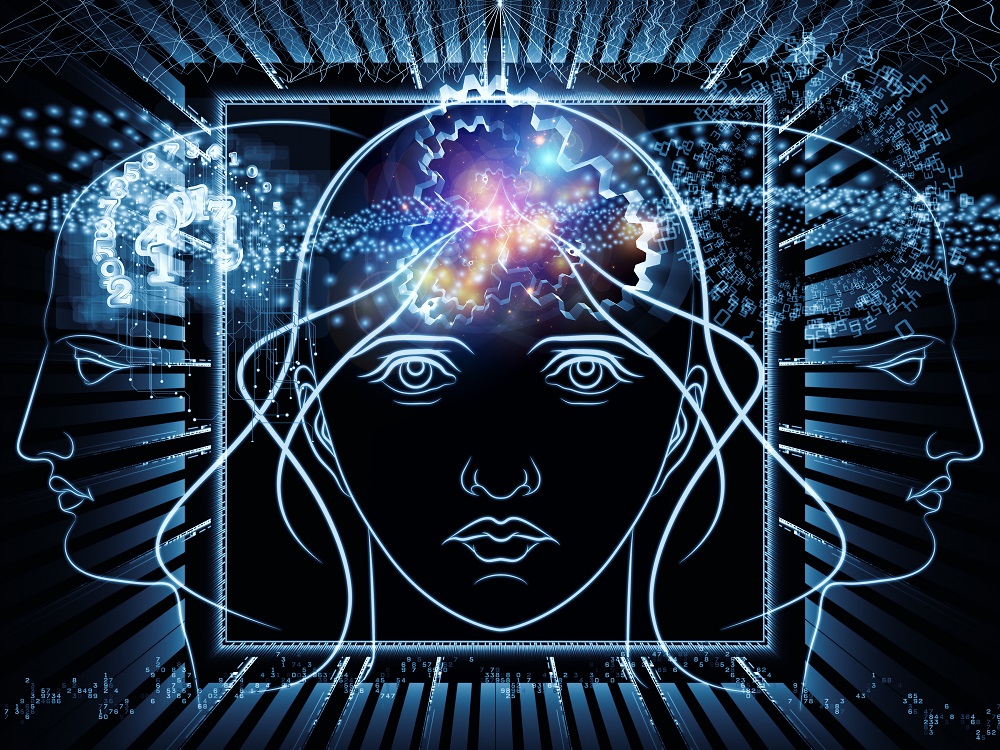
The need for speed is a hot topic among participants at this week’s AI Hardware Summit – larger AI language models, faster chips and more bandwidth for AI machines to make accurate predictions.
But some hardware startups are taking a throwback approach for AI computing to counter the more-is-better approach. Companies including Innatera, Rain Neuromorphics and others are creating silicon brains with analog circuitry to mimic brain functionality.
The brain is inherently analog, taking in raw sensory data, and these chipmakers are trying to recreate the way the brain’s neurons and synapses work in traditional analog circuitry.
Analog chips can be very good low-power sensing devices, especially for some sound and vision applications, said Kevin Krewell, an analyst at Tirias Research.
“Analog is a closer representation of how the brain acts using distributed memory cells to hold neuron weights or some other way to hold an analog weight,” Krewell said.
AI and machine learning rely mostly on digital chips on the edge or in data centers. But there is a place for analog chips on the edge, like in smartphones or cars, which need instant intelligence without sending data to the cloud, which is used to deliver AI services.
“We don’t aim to replace the complete AI pipeline,” said Sumeet Kumar, CEO of Innatera Nanosystems BV, which is based in Rijkswijk, Netherlands.
Innatera’s third-generation AI chip has 256 neurons and 65,000 synapses, which doesn’t sound like a lot compared to the human brain, which has 86 billion neurons, and operates at around 20 watts. But Kumar said it is possible to create a fully connected recurrent network on top, and the chip can operate on coin cell batteries.
The chip is being used by customers to run radar and audio applications, with performance that is competitive to other chips in the same class. The goal of the chip is to incorporate low levels of learning and inference on the device, which is considered a big challenge for AI among participants at the show.
“What we’re trying to do is, what we recognize is that when data is moving from a sensor into the cloud, it actually gets transformed at multiple stages by different kinds of AI. And what we very often see is customers doing low level sensor data handling in the cloud, which is completely unnecessary,” Kumar said.
The Innatera chip takes information coming in from a sensor, which is converted into spikes, and the content of the input is encoded into exactly when these spikes occur.
“That’s exactly how it happens in your brain. When you hear something, there are … tiny hair [cells] in your ear, which actually detects every frequency band and what is the energy within that band. And those hair [cells] will vibrate, produce spikes, which then go into the rest of your auditory cortex. Essentially, we’re following that exact same principle,” Kumar said.
Underlying that principle, inside of the neurons of brains there are calcium ions and low sodium ions, and those concentrations change over time. Innatera’s chip replicates that same sort of behavior using currents.
“We scale how much current is going into the neuron and coming out of the neuron. That’s how we are mimicking the brain,” Kumar said.
The idea isn’t to disrupt the current AI flow into the cloud, but to replace the current crop of AI chips on the edge that aren’t capable of making on-device decisions. The chip also reduces the process of converting analog signals to digital.
“You can’t really translate an analog signal over a long distance because then you actually have degradation. We avoid that by converting that analog signal into a spike,” Kumar said.
The foundation of AI today is based on simulating the action of the brain’s neurons using digital chips and techniques, which has been very successful. Based on the progress in Moore’s law, those digital circuits and networks have gotten larger and faster.
But analog has its problems. For example, it is harder to gain consistency across the analog chips with calibration issues like drift,
“Analog circuits and memory cells don’t scale like digital circuits. And most times analog eventually has to be converted to digital to interact with the rest of the system,” Krewell said.
To be sure, the concept of neuromorphic chips isn’t new. Companies like Intel and IBM have been developing brain-inspired chips, and universities are developing their own versions with analog circuitry. Intel and others have raised awareness of the difference between neuromorphic chips and conventional AI, but the startups felt the need to push out their products as AI compute requirements and power efficiency are growing at an unsustainable pace.
Another AI chip company, Rain Neuromorphics said its brain-mimicking chip would be used in particle accelerators at Argonne National Laboratory.
In a presentation at the AI Hardware Summit, the company didn’t provide much detail on how the chip would be used, but the company’s CEO, Gordon Wilson, said that the chip will act like silicon brains that would help the research lab study and draw conclusions on particle collisions.
The silicon brain will provide on-device intelligence to protect against sensor drift, which can cause faulty data to be fed to AI systems. The concept of sensor drift is similar to model drift in AI, in which bad data fed into a learning model can throw the AI system off course.
Wilson claimed the chip’s on-device capabilities are more power efficient compared to AI in the cloud.
“You need the ability to learn on the fly. You need the ability to train and fine tune against that sensor drift to maintain the performance of this system,” Wilson said.
The first iteration of the Rain chip “will essentially not look radically different from … other analog or mixing of chips,” Wilson said. But it will have the capacity to learn, which will unlock more value.
Wilson pointed to possibly different types of memory, like memristor circuits, providing the ability to learn. Memristors have been under development since the 1960s, and pursued by HP (which later became HPE) to use in a mega-computer called The Machine, but the technology still remains a novelty.
“Memristor serves as a memory resistor. It’s a resistor that can adjust its resistance. It’s used as an artificial synapse,” Wilson said. In a brain, synapses are not required to be perfect, and the requirements will be different for Rain’s memristors.
Venture capitalist Sam Altman, known for his work in AI as CEO of OpenAI, invested $25 million into Rain Neuromorphics earlier this year.
Source: https://www.hpcwire.com/2022/09/17/analog-chips-find-a-new-lease-of-life-in-artificial-intelligence/