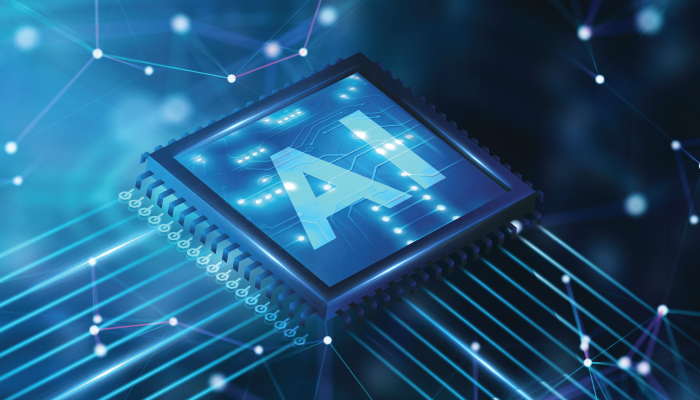
Through NLP-based interactive solutions, process automation tools, and business intelligence software, Machine Learning can help companies reach new heights. However, implementing this powerful technology within a solid management framework will spare businesses from several challenges in the future.
According to the 2022 State of AI report by CB Insights, US$15.1 billion in global funding was given to Artificial Intelligence (AI) startups in the first quarter of 2022. When used without reason, Machine Learning (ML) algorithms can, however, produce unfavorable outcomes.
Here are four challenges that businesses deploying ML-based systems may run into, as well as some tips on how to make the most of algorithms while avoiding pitfalls.
Also Read: Intelligent Automation – The Key to Digital Transformation Success
Identifying a Machine Learning Use Case
The first hurdles with adopting AI and ML are experienced by certain businesses even before they begin. Machine learning is a vast field that pervades most aspects of AI. It paves the way for a wide range of potential applications, from advanced data analytics and computer vision to Natural Language Processing (NLP) and Intelligent Process Automation (IPA).
A general rule of thumb for selecting a suitable ML use case is to “follow the money” in addition to the usual recommendations on framing the business goals – what companies expect Machine Learning to do for their business, like improving products or services, improving operational efficiency, and mitigating risk.
Depending on the size and industry, business leaders need to focus on the most strategic business operations and produce the maximum profit for their company. The elimination of enterprise weaknesses like process bottlenecks should be another key criterion for selection. Businesses can find them by conducting appropriate BPM investigations and KPI evaluations.
Also Read: Top 5 Justifications for Creating a Digital Workplace
Choosing the Right Data
The heart of Machine Learning is data.To be properly trained, ML systems require processing vast amounts of data. The caliber of the training procedure and the data sets themselves determine how reliable the results are.
Here are some suggestions to consider:
- Businesses must rely on skilled data scientists to choose appropriate data sources, whether they are gathered from internal or external corporate systems. To ensure that data is properly collected and stored, they must establish efficient data management and governance systems.
- Companies must choose a subset of core features from their datasets for the training phase to focus on the most important variables and ignore unnecessary metrics, facilitating result interpretation.
- Enterprises must train their Machine Learning system with several additional data samples to monitor and improve it under various scenarios while avoiding overfitting problems, which occur when algorithms are optimized for some data sets but perform poorly with others.
ML in Combination with Human Talent
Even after training for data analysis, Machine Learning algorithms may still behave in an unpredictable manner. This ambiguity may be a problem if using AI in decision-making results in unanticipated results. This is why continuous human supervision should always be there for ML-based analysis. Skilled professionals should keep an eye on how the ML system is performing in real-world situations and adjust its parameters using additional training datasets that include new trends or scenarios. Decision-making should not be ML-imposed; it should be ML-driven. The recommendations for the system should not be taken at face value but rather thoroughly examined.
Unfortunately, due to a shortage of ML experts, merging algorithms and human expertise continues to be difficult. The severity of the skill gap worries decision-makers all over the world. This problem can be solved with investments in employee training and collaborations with other businesses that are interested in implementing machine learning.
Overcoming Resistance to Change
The biggest obstacles to the adoption of ML may be resistance to change, corporate inertia, and a lack of preparation. The biggest obstacle to deploying AI-related technologies is corporate culture. Top management is often reluctant to take investment risks, and employees worry about losing their jobs. Businesses must start with small-scale ML use cases that demand realistic investments to achieve quick wins and persuade executives in order to assure stakeholder and employee buy-in. By providing workshops, corporate training, and other incentives, they can promote innovation and digital literacy.
In order to oversee ML implementation across the organization, including the technological and operational adjustments needed to integrate these tools into the enterprise workflow and software ecosystem, it is also critical to establish centers of excellence.
Check Out The New Enterprisetalk Podcast. For more such updates follow us on Google News Enterprisetalk News.