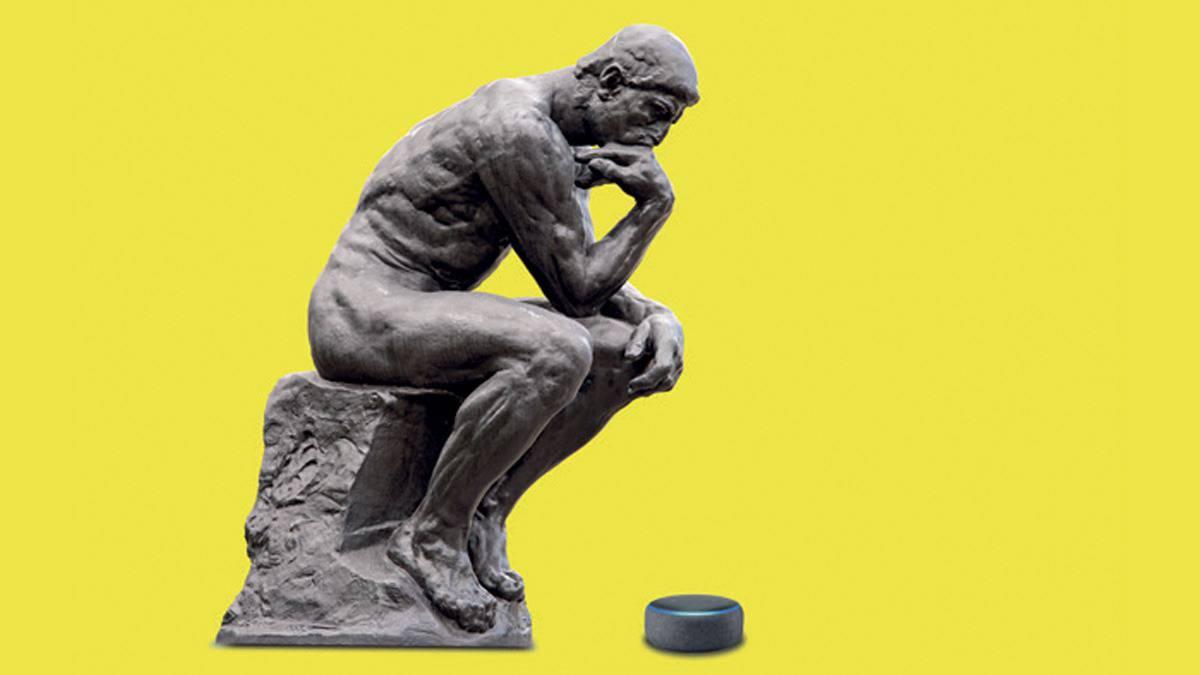
Amazon terminated its recruiting tool in 2018 after it was found to show a bias against women. So, why did the artificial intelligence (AI) tool not like women candidates? When the engineers dug into it, they found that the AI was trained using data from a time when the tech industry was dominated by men. And in doing so, the AI had ‘learnt’ that male candidates were preferable. Not only that, its machine learning (ML) model had learnt to penalise resumes with words like “women’s” as in “women’s chess club”. And that was the reason why it recommended only male candidates.While Amazon stopped using the tool once the issue came to light, it has become a prime example of how not to deploy AI systems. But even five years later, the relevance of this incident has only grown. Sample this: per Accenture’s 2022 Tech Vision Research report, only 35 per cent of users globally trust how AI is implemented across companies. And about 77 per cent believe that companies must be held responsible for any misuse of AI.
And while big tech companies have more experience working with developing and potentially harmful tech, the responsible use of AI within the Indian start-up ecosystem poses a big question too.
For context, India’s start-up ecosystem is the third largest after the US and China. Per estimates by Nasscom and consultancy Zinnov, there were about 3,000 tech start-ups in India in 2021, with the maximum number from the field of AI (1,900).
But are tech start-ups aware of the responsibility they bear when it comes to deploying AI/ML? Inculcating the values of Responsible AI (RAI) from the beginning is important, say experts. “Sometimes, early-stage start-ups don’t necessarily pay heed to compliance because they have so much to do,” says Srinivas Rao Mahankali, CEO of start-up innovation centre T-Hub, adding that any such omission may come back to bite them later.
Conversations around RAI don’t happen at the early stages, says an investor who has invested in many start-ups, on condition of anonymity. A lot of times, founders are aware of the pitfalls of such tech being misused, but they are inadequately equipped to avoid them, says the investor. Stakeholders also point out that while there is knowledge of such matters at the executive level, it needs to trickle down the corporate pyramid to avoid their harmful effects in real-life situations. But then, how does one get started?
The intelligence impact
Let us start with the basics. What is RAI? Professional services firm Accenture defines it as the practice of designing and deploying AI with good intentions—to empower employees and businesses and fairly impact customers—and scale AI with confidence and trust.
Also called trustworthy or ethical AI, RAI has many applications. For example, in India, RAI’s use can be found in retail preferences, inventory management, cybersecurity, healthcare, banking, etc.
“In healthcare, AI is used to provide insights for medical diagnosis, and identify complex health patterns. In such cases, safety, bias and explainability (the concept that an AI/ML model and its output can be explained in a way that “makes sense” to a human being) are major concerns. In banking, AI is deployed to detect fraud and analyse user behaviour. This too raises fairness and privacy issues,” says Sachin Lodha, Chief Scientist at TCS Research.
These use cases reveal that RAI affects not just enterprises, but society at large, especially the end users. Directly or indirectly, AI has the power to reshape resource allocation and policy decisions, among other things. Therefore, it is imperative to assess how they can be made more transparent, fair, accurate, risk-free and free of biases.
Some simple steps that companies can take to build RAI include awareness around capacity building, assessing impacts, creating prototypes and testing it on various metrics like behavioural patterns, fairness, explainability, and more. And then working to filter out the biased patterns that are observed. Simply put, RAI is about designing systems that condense what has happened to identify issues that can arise and then take proactive steps to eliminate them. However, one step that start-ups need to be careful about is the usage of data in their models.
Typically, an AI is fed a lot of data, and it calculates based on that, says Achyut Chandra, Manager and Lead of HCL’s Open Innovation. That’s why, he says, being specific is vital. “Considering the feature we are capturing from the data sets is very important,” he adds.
This is exactly what happened in Amazon’s case. It had been fed data from a time when the industry was dominated by male candidates. And the AI was showing the results based on the inputs it had got. To solve this, Ankit Bose, Nasscom’s AI Head, suggests that start-ups should try to understand the biases in data so well that they start creating their own data sets at the source level.
The self-regulation key
Currently, there are many tools available (from Microsoft, Google, etc.) to check the performance of AI systems, but there is no regulatory oversight. And that is why, experts believe that companies, new and old, need to put more thought into self-regulation.
For instance, tech giant TCS’ strategy has been to do pilots with many players, along with regular audits of its processes to refine its RAI models. “This has created an API-fied version of a tool that is extensible, fungible and applies to different types of AI models like computer vision, natural language processing, sequence to sequence models, etc.,” says Lodha.
Another way to carry out checks and balances is through thorough audits. “A regular audit of their processes and timely validation of compliance to any changes in standards in the RAI space is required,” says Lodha. He also cites the European Commission’s High-Level Expert Group on AI’s ethics guidelines, or America’s National AI Initiative’s Strategic Pillars as good reference points to start with.
Tech giants apart, there are also some well-known start-ups that have carved out ways to implement RAI. Monish Darda, CTO and Co-founder of SaaS unicorn Icertis—that provides contract lifecycle management (CLM) solutions—says a few years ago, they integrated Explainable AI and Ethical AI into their AI systems. Explainable AI allows Icertis to explain the results produced by their AI, enabling the correlation between the result and the data that produced it. “This has worked very well for us because we get to know how we arrived at a prediction and what we missed,” he says. Ethical AI, meanwhile, helps Icertis assure its users and customers that the data used in training its AI tools is sourced with permission and for the purpose it was intended. The platform also ensures that the data set is unbiased by picking up a reasonable sample of the data after considering aspects like geographies, culture, etc.
Not only that, there is a huge thrust on engaging people from different domains to make fair and just AI systems. “To push the positive applications of AI, it is critical to continually ask questions, engage experts—such as software developers, data scientists, legal experts, the founders, etc.—and progress together,” says a Google spokesperson.
“Awareness is one of the biggest pillars. We need people who understand RAI,” says Bose, adding, when people understand RAI, they will be able to identify the ways in which AI models can interpret data in the same way as humans, and develop resources that can reverse-engineer issues when they arise.
It’s not magic
Icertis’ Darda says that RAI is not magic, nor is it a destination. The need for a company to implement AI responsibly is a continuous one, he asserts, adding that it will take around 10-15 years to crack RAI. “But we will come closer to figuring out how to remove biases,” he says. From his own experience, Darda echoes T-Hub CEO Rao’s point when he says that sometimes entrepreneurs are so excited about tech that they forget about the way they treat their data and algorithms. Thus, brushing aside the topic of RAI is not an option. Start-ups need to lead from the front because by not following the appropriate standards, they are putting users in peril.
Akbar Mohammed, Chief Data Scientist at data intelligence company Fractal Analytics, cites the example of AI’s use cases in mental healthcare as a word of caution. “Today we have AI that can detect if you have a mental health issue based on how you have conversations with your friends, what kind of news you browse, etc. And that intelligence can be used both to provide support if you need it, or be abused. Bad elements can use it to reduce your potential employability prospects in the market.”
So what is the way forward? “It’s hard!” admits Shantanu Narayen, Chairman and CEO of software firm Adobe. Darda adds that thinking about RAI from the designing phase is something that will make or break a company. Responsible deployment of tech is as important as product market fit, customers, finance and more, he says. “You have to think about the unintended consequences and the biases that can emerge. I would advise start-ups to have broader and powerful data sets, do a lot of testing, and run a lot of pilots,” adds Narayen.
The bottom line that all the stakeholders agree on lies in intent and the purpose with which you build an AI model. And start-ups that are dealing with large processes, data and other information must get it right from the start. Even now, it is not too late. Why? Because it all starts with asking a simple question: am I implementing AI responsibly?
@Bhavyakaushal2
Source: https://news.google.com/__i/rss/rd/articles/CBMiogFodHRwczovL3d3dy5idXNpbmVzc3RvZGF5LmluL21hZ2F6aW5lL3RlY2hub2xvZ3kvc3RvcnkvYmVpbmctc2Vuc2libGUtd2l0aC1haS13aHktdGVjaC1jb21wYW5pZXMtbmVlZC10by1iZS1jYXJlZnVsLXdpdGgtYXJ0aWZpY2lhbC1pbnRlbGxpZ2VuY2UtMzU4Mjk5LTIwMjItMTItMzDSAaYBaHR0cHM6Ly93d3cuYnVzaW5lc3N0b2RheS5pbi9hbXAvbWFnYXppbmUvdGVjaG5vbG9neS9zdG9yeS9iZWluZy1zZW5zaWJsZS13aXRoLWFpLXdoeS10ZWNoLWNvbXBhbmllcy1uZWVkLXRvLWJlLWNhcmVmdWwtd2l0aC1hcnRpZmljaWFsLWludGVsbGlnZW5jZS0zNTgyOTktMjAyMi0xMi0zMA?oc=5