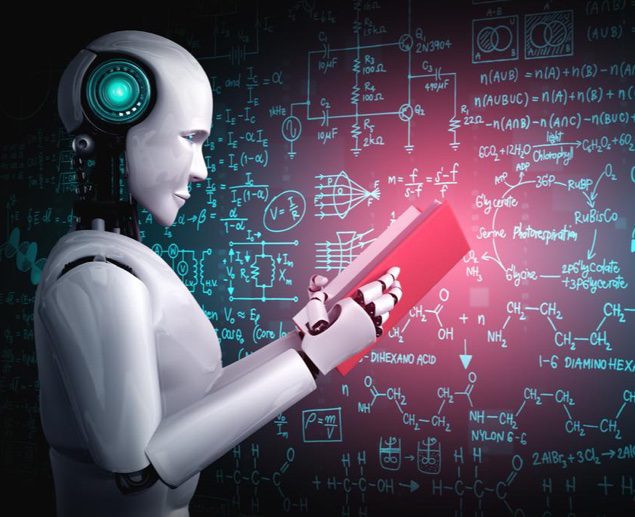
By any measure, artificial intelligence (AI) has become big business.
According to Gartner, customers worldwide will spend $62.5 billion on AI software in 2022. And it notes that 48 percent of CIOs have either already deployed some sort of AI software or plan to do so within the next twelve months.
All that spending has attracted a huge crop of startups focused on AI-based products. CB Insights reported that AI funding hit $15.1 billion in the first quarter of 2022 alone. And that came right after a quarter that saw investors pour $17.1 billion into AI startups. Given that data drives AI, it’s no surprise that related fields like data analytics, machine learning and business intelligence are all seeing rapid growth.
But what exactly is artificial intelligence? And why has it become such an important — and lucrative — part of the technology industry?
Also see: Top AI Software
And: What is Generative AI?
What Is Artificial Intelligence?
In some ways, artificial intelligence is the opposite of natural intelligence. If living creatures can be said to be born with natural intelligence, man-made machines can be said to possess artificial intelligence. So from a certain point of view, any “thinking machine” has artificial intelligence.
And in fact, one of the early pioneers of AI, John McCarthy, defined artificial intelligence as “the science and engineering of making intelligent machines.”
In practice, however, computer scientists use the term artificial intelligence to refer to machines doing the kind of thinking that humans have taken to a very high level.
Computers are very good at making calculations — at taking inputs, manipulating them, and generating outputs as a result. But in the past they have not been capable of other types of work that humans excel at, such as understanding and generating language, identifying objects by sight, creating art, or learning from past experience.
But that’s all changing.
Today, many computer systems have the ability to communicate with humans using ordinary speech. They can recognize faces and other objects. They use machine learning techniques, especially deep learning, in ways that allow them to learn from the past and make predictions about the future.
So how did we get here?
Also see: How AI is Altering Software Development with AI-Augmentation
A Short History of Artificial Intelligence
Many people trace the history of artificial intelligence back to 1950, when Alan Turing published “Computing Machinery and Intelligence.” Turing’s essay began, “I propose to consider the question, ‘Can machines think?’” It then laid out a scenario that came to be known as a Turing Test. Turing proposed that a computer could be considered intelligent if a person could not distinguish the machine from a human being.
In 1956, John McCarthy and Marvin Minsky hosted the first artificial intelligence conference, the Dartmouth Summer Research Project on Artificial Intelligence (DSRPAI). It convinced computer scientists that artificial intelligence was an achievable goal, setting the foundation for several decades of further research. And early forays into AI technology developed bots that could play checkers and chess.
The 1960s saw the development of robots and several problem-solving programs. One notable highlight was the creation of ELIZA, a program that simulated psychotherapy and provided an early example of human-machine communication.
In the 1970s and 80s, AI development continued but at a slower pace. The field of robotics in particular saw significant advances, such as robots that could see and walk. And Mercedes-Benz introduced the first (extremely limited) autonomous vehicle. However, government funding for AI research decreased dramatically, leading to a period some refer to as the “AI winter.”
Interest in AI surged again in the 1990s. The Artificial Linguistic Internet Computer Entity (ALICE) chatbot demonstrated that natural language processing could lead to human-computer communication that felt far more natural than what had been possible with ELIZA. The decade also saw a surge in analytic techniques that would form the basis of later AI development, as well as the development of the first recurrent neural network architecture. This was also the decade when IBM rolled out its Deep Blue chess AI, the first to win against the current world champion.
The first decade of the 2000s saw rapid innovation in robotics. The first Roombas began vacuuming rugs, and robots launched by NASA explored Mars. Closer to home, Google was working on a driverless car.
The years since 2010 have been marked by unprecedented increases in AI technology. Both hardware and software developed to a point where object recognition, natural language processing, and voice assistants became possible. IBM’s Watson won Jeopardy. Siri, Alexa, and Cortana came into being, and chatbots became a fixture of modern retail. Google DeepMind’s AlphaGo beat human Go champions. And enterprises in all industries have begun deploying AI tools to help them analyze their data and become more successful.
Now AI is truly beginning to evolve past some of the narrow and limited types into more advanced implementations.
Also see: The History of Artificial Intelligence
Types of AI
Different groups of computer scientists have proposed different ways of classifying the types of AI. One popular classification uses three categories:
- Narrow AI does one thing really well. Apple’s Siri, IBM’s Watson, and Google’s AlphaGo are all examples of Narrow AI. Narrow AI is fairly common in the world today.
- General AI is a theoretical form of AI that performs most intellectual tasks on par with a human. Examples from popular movies might include HAL from “2001: A Space Odyssey” or J.A.R.V.I.S. from “Iron Man.” Many researchers are currently working on developing general AI.
- Super AI, which is also still theoretical, has intellectual capacities that far outstrip those of humans. This kind of artificial intelligence is not yet close to becoming a reality.
Another popular classification uses four different categories:
- Reactive machines take an input and deliver an output, but they do not have any memory or learn from past experience. The bots you can play against in many video games are good examples of reactive machines.
- Limited memory machines can look a little way back into the past. Many of the vehicles on the road today have advanced safety features that would fall into this category. For example, if your car issues a backup warning when a vehicle or person is about to pass behind your car, it is using a limited set of historical data to come to conclusions and deliver outputs.
- Theory of mind machines are aware that human beings and other entities exist and have their own independent motivations. Most researchers agree that this kind of AI has not yet been developed, and some researchers say that we should not attempt to do so.
- Self-aware machines are aware of their own existence and identities. Although a few researchers claim that self-aware AI exists today, only a handful of people share this opinion. Developing self-aware AI is highly controversial.
While these classifications are interesting from a theoretical standpoint, most organizations are far more interested in what they can do with AI. And that brings us to the aspect of AI that is generating a lot of revenue — the AI use cases.
Also see: Three Ways to Get Started with AI
AI Use Cases
The possible AI use cases and applications for artificial intelligence are limitless. Some of today’s most common AI use cases include the following:
- Recommendation engines — Whether you’re shopping for a new sweater, looking for a movie to watch, scrolling through social media or trying to find true love, you’re likely to encounter an AI-based algorithm that makes suggestions. Most recommendation engines use machine learning models to compare your characteristics and historical behavior to that of people around you. The models can be very good at identifying preferences even when users aren’t aware of those preferences themselves.
- Natural language processing — Natural language processing (NLP) is a broad category of AI that encompasses speech-to-text, text-to-speech, keyword identification, information extraction, translation and language generation. It allows humans and computers to interact through ordinary human language (audio or typed), rather than through programming languages. Because many NLP tools incorporate machine learning capabilities, they tend to improve over time.
- Sentiment analysis — AI can not only understand human language, it can also identify the emotions underpinning human conversation. For example, AI can analyze thousands of tech support conversations or social media interactions and identify which customers are experiencing strong positive or negative emotions. This type of analysis can allow customer support teams to focus on customers that might be at risk of defecting and/or extremely enthusiastic supporters who could be encouraged to become advocates for the brand.
- Voice assistants — Many of us interact with Siri, Alexa, Cortana or Google on a daily basis. While we often take these assistants for granted, they incorporate advanced AI techniques, including natural language processing and machine learning.
- Fraud prevention — Financial services companies and retailers often use highly advanced machine learning techniques to identify fraudulent transactions. They look for patterns in financial data, and when a transaction looks abnormal or fits a known pattern of fraud, they issue alerts that can stop or mitigate criminal activity.
- Image recognition — Many of us use AI-based facial recognition to unlock our phones. This kind of AI also enables autonomous vehicles and allows for automated processing of many health-related scans and tests.
- Predictive maintenance — Many industries like manufacturing, oil and gas, transportation, and energy rely heavily on machinery, and when that machinery experiences downtime, it can be extremely costly. Firms are now using a combination of object recognition and machine learning techniques to identify in advance when equipment is likely to break down so that they can schedule maintenance at a time when it minimizes disruptions.
- Predictive and proscriptive analytics — Predictive algorithms can analyze just about any kind of business data and use that as the basis for forecasting likely future events. Prescriptive analytics, which is still in its infancy, goes a step further and not only makes a forecast, but also offers recommendations as to what organizations should do to prepare for likely future events.
- Autonomous vehicles — Most of the vehicles in production today have some autonomous features, such as parking assistance, lane centering and adaptive cruise. And while they are still expensive and relatively rare, fully autonomous vehicles are already on the road, and the AI technology that powers them is getting better and less expensive every day.
- Robotics — Industrial robots were one of the earliest implementations of AI, and they continue to be an important part of the AI market. Consumer robots, such as robot vacuum cleaners, bartenders, and lawn mowers, are becoming increasingly commonplace.
Of course, these are just some of the more widely known use cases for AI. The technology is seeping into daily life in so many ways that we often aren’t fully aware of them.
Also see: Best Machine Learning Platforms
The Future of AI
So where is the future of AI? Clearly it is reshaping consumer and business markets.
The technology that powers AI continues to progress at a steady rate. Future advances like quantum computing may eventually enable major new innovations, but for the near term, it seems likely that the technology itself will continue along a predictable path of constant improvement.
What’s less clear is how humans will adapt to AI. This question poses questions that loom large over human life in the decades ahead.
Many early AI implementations have run into major challenges. In some cases, the data used to train models has allowed bias to infect AI systems, rendering them unusable.
In many other cases, business have not seen the financial results they hoped for after deploying AI. The technology may be mature, but the business processes surrounding it are not.
“The AI software market is picking up speed, but its long-term trajectory will depend on enterprises advancing their AI maturity,” said Alys Woodward, senior research director at Gartner.
“Successful AI business outcomes will depend on the careful selection of use cases,” Woodware added. “Use cases that deliver significant business value, yet can be scaled to reduce risk, are critical to demonstrate the impact of AI investment to business stakeholders.”
Organizations are turning to approaches like AIOps to help them better manage their AI deployments. And they are increasingly looking for human-centered AI that harnesses artificial intelligence to augment rather than to replace human workers.
In a very real sense, the future of AI may be more about people than about machines.
Also see: The Future of Artificial Intelligence
For more information about artificial intelligence, also see:
What is Generative AI?
The AI Market: An Overview
Cloud and AI Combined: Revolutionizing Tech