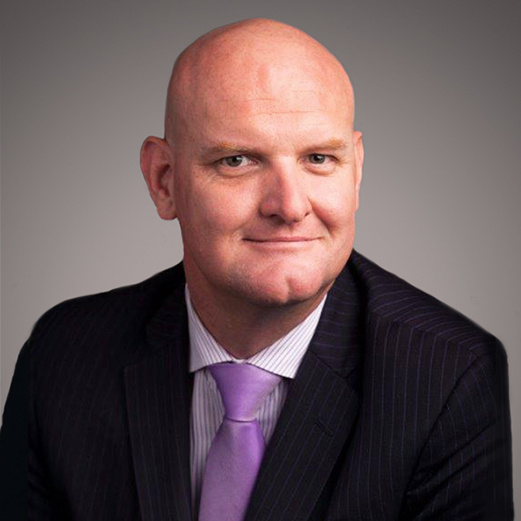
Enterprise security is in the midst of a fundamental and necessary shift in direction. A significant reskilling movement is underway, but not everyone is fully aware of what is happening and why.
Of course, security teams adjust all the time to new threats. But increasingly they are also having to adapt the scope and complexity of security to the increasingly digital-driven businesses they support. The number of issues to consider is daunting and the pace can be overwhelming.
Hiring more analysts for your Cyber Defense Operations wouldn’t solve the problem, even if you could find them. Based on my own experience and discussions with hundreds of the world’s most complex organizations, I’ve found that through the intelligent application of artificial intelligence (AI) and machine learning (ML), it’s possible to make good progress and get ahead of new threats.
Increase Automation Toolkit for Security Teams
To begin to understand this evolution, step back for a moment and consider the role of security executives and their teams. The job of a security executive is not to just purchase a bunch of security tools and call that a “strategy.” Their purpose is to develop a comprehensive security posture for the enterprise that is built around skilled security personnel able to execute processes that use those tools to protect and nurture the organization. CISOs must grow and adapt their security capacity to keep up with the pace of digital transformation.
The capacity equation is not:
- Capacity = Size of Security team + number of security tools
But rather,
- Capacity = (Size of security team + Number of security tools + data foundation) * automation
CISOs should amplify the power of their teams by building a comprehensive data foundation to describe their environments and then expand how they automate analysis, hunting, and response using AI and ML, powered by that data.
AI/ML Reveal the Invisible so Security Teams Can Act Immediately
Today, all security functions include some version of security analytics. When you think about large-scale security incident and event monitoring (SIEM) platforms, they all ingest data from across a wide variety of areas of interest to the organization, then try to correlate that data to identify and prioritize threats. These are good first steps toward automating an intelligent security capability, but a SIEM is only as useful as its data foundation, and an organization needs to be able to extract much deeper insights and move to a more predictive state.
With that data available to analyze, you can move beyond the narrow event focus that limits many security teams, to more quickly and efficiently respond to threats you know about. But what about all the unknown threats? AI and ML learning models make it possible for security teams to apply techniques like neural networking, entity, and clustering analysis that make it possible to identify things they haven’t seen before.
When we say “fast,” we mean machine speed. Threat actors, even unsophisticated ones, can change their techniques quickly, recognizing your playbook and adjusting tactics accordingly. This is the challenge that security teams face every day. We need to train machines to anticipate potential changes in threat tactics. That is becoming possible now that data science techniques are being applied to security use cases, resulting in a shift to a more predictive capability.
Security Teams Are Business Assets
All that data about security is really data about business activity and what’s happening in that environment. It is a reflection of how the organization operates. With this insight, the security team is fast becoming an intelligence capability for the organization — and is uniquely positioned to separate fiction from reality. Armed with this information, the organization can start making smarter, data-driven decisions not only about vulnerabilities and attacks but also about how it conducts business operations.
For example, consider that a line of business has mapped out a process for working with a partner. There is an assumption that the defined process is the de facto way things work. However, how that process is executed by the front-line staff may not be as defined or there may be a subset of users that do something different, exposing the organization to undue risk. A security team armed with the right insights can enable the organization to manage this previously unknown or unseen risk.
This is an important change for security teams, many of which have reacted from a defensive posture until recently. Being in a position to help the organization identify broken processes and make smarter decisions about how it conducts business is a sure sign of a security team that is harnessing the value of the data it has at hand and is evolving its role in the organization.
Who Watches the Watchers?
AI and ML are not magic. They are technological advancements as vulnerable to misuse and damage as any other. Even as we embrace AI/ML, we need to be certain of the validity of the models and be assured that the results are what we expect. AI/ML comes with its own need for risk management. Just imagine the harm that might come if the data that is used to train the model is tampered with or poisoned?
As companies expand their use of AI/ML across their business operations they will need to address the vulnerabilities of these capabilities, as well to ensure the model is providing the correct outcomes.
Are We Facing a Skills Shortage or Just the Wrong Allocation of Security Experts?
While there’s a lot of talk about the industry’s skill shortage, much of that shortage has emerged because the nature of threats has changed. Security teams can only use the tools and processes available to them. They can’t create the automated security capability their organizations need if they are stuck with the manual response and threat-hunting tools of yesterday.
If we are serious about driving down the mean-time-to-detect, mean-time-to-contain, and other critical measures of security team success, then we need to shift focus. We can’t keep throwing people at the problem — we need to act at machine speed, and this won’t happen overnight. Organizations need to act now to develop capabilities by building out their data science functions, which might mean reskilling some security staff. It might also mean working with the organization’s data science team to build out needed capabilities in data science.
Where Do You Start?
Let’s take a step back from the technology itself and examine the true asset here: the data. Security teams have access to vast amounts of data derived from all the different tools they’ve deployed already. The key is bringing all these disparate data sets together and generating insights from what otherwise would have been missed should these data sets be left in isolation.
How do you identify patterns in the environment when you are looking holistically across it? Due to the exponential growth of data and cost of storage then becoming a blocker, the security information and event management (SEIM) system will often only have a subset of what you really need. The trick is to land all relevant data from all sources, either central or distributed, that allows a data science team to apply their skills and generate the insights, and furthermore, leverage the capabilities of AI and ML in order to build predictive capabilities.
The key is building the right skill sets. The data science team can’t do this alone, and cyber specialists will need to work hand in hand with the data scientists. Over time, these data scientists will become cyber experts themselves, and vice-versa. This is exactly how security teams will evolve in the future.
AI and ML excel at enabling you to identify patterns in disparate data sets; things that have been missed in the past because we haven’t been able to analyze them fast enough. It’s time to apply the smarts of cyber experts to building machine-aided capabilities that will enable security teams to act at machine speed — and help business leaders make the best decisions.
Feature image via Pixabay.